AlphaFold, a groundbreaking AI system developed by DeepMind, has revolutionized the field of structural biology by accurately predicting protein structures. As we look to the future, the potential applications and advancements of AlphaFold technology are poised to transform numerous scientific and medical domains. By providing unprecedented insights into protein folding, AlphaFold is expected to accelerate drug discovery, enhance our understanding of diseases, and facilitate the development of novel therapeutics. Furthermore, the integration of AlphaFold with other emerging technologies could lead to innovative solutions in biotechnology, personalized medicine, and synthetic biology. As researchers continue to refine and expand upon this technology, AlphaFold’s impact on science and healthcare is likely to grow, offering a glimpse into a future where complex biological challenges are addressed with greater precision and efficiency.
Advancements In Protein Structure Prediction
The field of protein structure prediction has witnessed a transformative leap with the advent of AlphaFold technology, a groundbreaking development that has redefined the boundaries of computational biology. AlphaFold, developed by DeepMind, has demonstrated unprecedented accuracy in predicting protein structures, a task that has long posed significant challenges to scientists. As we look to the future, the potential advancements in AlphaFold technology promise to further revolutionize our understanding of biological processes and accelerate the pace of scientific discovery.
To appreciate the future trajectory of AlphaFold, it is essential to understand its current capabilities. AlphaFold’s success lies in its ability to predict the three-dimensional structure of proteins from their amino acid sequences with remarkable precision. This achievement is particularly significant because the structure of a protein largely determines its function, and understanding this relationship is crucial for numerous applications, including drug discovery and disease research. By providing accurate models of protein structures, AlphaFold has already begun to impact these fields, offering insights that were previously unattainable through experimental methods alone.
Looking ahead, one of the most promising areas for advancement in AlphaFold technology is its integration with other computational and experimental techniques. By combining AlphaFold’s predictive power with methods such as cryo-electron microscopy and nuclear magnetic resonance spectroscopy, researchers can validate and refine protein models, leading to even greater accuracy and reliability. This synergy between computational predictions and experimental validation is expected to enhance our understanding of complex biological systems, paving the way for novel therapeutic interventions.
Moreover, the future of AlphaFold technology is likely to be characterized by its application to increasingly complex protein structures and interactions. While AlphaFold has excelled in predicting the structures of individual proteins, the challenge of understanding protein complexes and their dynamic interactions remains. Future iterations of AlphaFold could incorporate machine learning algorithms capable of modeling these intricate interactions, providing a more comprehensive view of cellular processes. This advancement would be particularly beneficial in the study of diseases such as cancer and neurodegenerative disorders, where protein interactions play a critical role.
In addition to its scientific applications, the future of AlphaFold technology holds promise for democratizing access to protein structure prediction. As the technology becomes more refined and accessible, it is anticipated that researchers across the globe, regardless of their resources, will be able to leverage AlphaFold for their investigations. This democratization could lead to a surge in collaborative research efforts, fostering innovation and accelerating the pace of discovery in the life sciences.
Furthermore, the continued development of AlphaFold technology is expected to drive advancements in artificial intelligence and machine learning. The algorithms and computational techniques underpinning AlphaFold have already set new benchmarks in these fields, and their evolution will likely inspire further breakthroughs. As AI continues to evolve, it will not only enhance our ability to predict protein structures but also open new avenues for exploring other complex biological phenomena.
In conclusion, the future of AlphaFold technology is poised to bring about significant advancements in protein structure prediction, with far-reaching implications for science and medicine. By integrating with experimental techniques, tackling complex protein interactions, democratizing access, and driving AI innovation, AlphaFold is set to remain at the forefront of computational biology. As researchers continue to explore its potential, AlphaFold promises to unlock new frontiers in our understanding of life at the molecular level, heralding a new era of scientific discovery.
Impact On Drug Discovery And Development
The advent of AlphaFold technology has marked a significant milestone in the field of structural biology, with profound implications for drug discovery and development. AlphaFold, an artificial intelligence system developed by DeepMind, has demonstrated an unprecedented ability to predict protein structures with remarkable accuracy. This breakthrough addresses a long-standing challenge in biology, as understanding protein structures is crucial for elucidating their functions and interactions. Consequently, the impact of AlphaFold on drug discovery and development is poised to be transformative, offering new avenues for innovation and efficiency in the pharmaceutical industry.
To begin with, the ability of AlphaFold to predict protein structures with high precision accelerates the initial stages of drug discovery. Traditionally, determining the three-dimensional structure of proteins has been a labor-intensive and time-consuming process, often involving techniques such as X-ray crystallography or cryo-electron microscopy. These methods, while effective, can take months or even years to yield results. In contrast, AlphaFold can predict protein structures in a matter of days, significantly reducing the time required to identify potential drug targets. This rapid turnaround not only expedites the research process but also allows scientists to explore a broader range of proteins, including those that were previously considered too challenging to study.
Moreover, AlphaFold’s impact extends beyond the speed of discovery. By providing accurate structural predictions, it enhances the ability of researchers to design drugs that precisely target specific proteins. This precision is crucial in developing therapies that are both effective and have minimal side effects. For instance, in the case of diseases caused by misfolded proteins, such as Alzheimer’s or cystic fibrosis, AlphaFold can aid in designing molecules that stabilize the correct protein conformation, potentially leading to more effective treatments. Furthermore, the technology’s ability to predict protein-protein interactions opens new possibilities for targeting complex biological pathways, which are often involved in multifactorial diseases like cancer.
In addition to its direct applications in drug design, AlphaFold also holds promise for improving the efficiency of clinical trials. By providing detailed insights into protein structures, it can help identify biomarkers that predict patient responses to specific treatments. This capability enables the stratification of patients in clinical trials, ensuring that therapies are tested on those most likely to benefit. Consequently, this targeted approach can lead to more successful trials, reducing the time and cost associated with bringing new drugs to market.
While the potential of AlphaFold is immense, it is important to acknowledge the challenges that remain. The technology is not infallible and may struggle with certain classes of proteins or complex molecular assemblies. Additionally, integrating AlphaFold predictions into existing drug discovery pipelines requires careful validation and collaboration between computational and experimental scientists. Nevertheless, the ongoing refinement of AlphaFold and its integration with other computational tools promise to address these challenges, further enhancing its utility in drug development.
In conclusion, AlphaFold technology represents a paradigm shift in the field of drug discovery and development. By dramatically accelerating the process of protein structure determination and enabling more precise drug design, it holds the potential to revolutionize the pharmaceutical industry. As researchers continue to harness its capabilities and address its limitations, AlphaFold is set to play a pivotal role in the development of next-generation therapies, ultimately improving patient outcomes and advancing the frontiers of medicine.
Integration With Genomic Data For Personalized Medicine
The advent of AlphaFold technology has marked a significant milestone in the field of structural biology, offering unprecedented insights into protein folding and structure prediction. As we look to the future, the integration of AlphaFold with genomic data presents a promising frontier for personalized medicine. This convergence of technologies holds the potential to revolutionize how we understand and treat diseases at an individual level, paving the way for more precise and effective therapeutic interventions.
AlphaFold, developed by DeepMind, has demonstrated remarkable accuracy in predicting protein structures, a task that has historically been fraught with complexity and uncertainty. By leveraging deep learning algorithms, AlphaFold can predict the three-dimensional shapes of proteins based solely on their amino acid sequences. This capability is crucial because the structure of a protein largely determines its function and role in biological processes. Consequently, understanding protein structures can provide critical insights into the mechanisms of diseases and the development of targeted treatments.
In parallel, the field of genomics has made significant strides, particularly with the decreasing cost and increasing accessibility of whole-genome sequencing. This has led to an explosion of genomic data, offering a comprehensive view of the genetic variations that contribute to individual health and disease susceptibility. However, the challenge lies in interpreting this vast amount of data to derive meaningful insights that can inform clinical decision-making.
The integration of AlphaFold with genomic data offers a promising solution to this challenge. By combining protein structure predictions with genomic information, researchers can gain a deeper understanding of how genetic variations affect protein function and, consequently, disease phenotypes. This integrated approach can help identify novel biomarkers for disease, predict individual responses to drugs, and uncover new therapeutic targets.
Moreover, this integration can facilitate the development of personalized medicine strategies. Personalized medicine aims to tailor medical treatment to the individual characteristics of each patient, taking into account their genetic makeup, environment, and lifestyle. By understanding the specific protein structures associated with a patient’s genetic profile, clinicians can design more effective treatment plans that target the underlying molecular mechanisms of disease. This could lead to improved outcomes and reduced adverse effects, as treatments are more precisely aligned with the patient’s unique biological context.
Furthermore, the integration of AlphaFold with genomic data can accelerate drug discovery and development. Traditional drug discovery processes are often time-consuming and costly, with a high rate of failure. By providing detailed insights into protein structures, AlphaFold can help identify potential drug targets more efficiently. When combined with genomic data, researchers can prioritize targets that are most relevant to specific patient populations, thereby increasing the likelihood of developing successful therapies.
In conclusion, the future of AlphaFold technology lies in its integration with genomic data, offering transformative potential for personalized medicine. This synergy promises to enhance our understanding of disease mechanisms, improve the precision of medical treatments, and accelerate the development of new therapies. As these technologies continue to evolve, they hold the promise of ushering in a new era of healthcare, where treatments are not only more effective but also tailored to the unique genetic makeup of each individual. The journey towards this future is complex and requires continued collaboration across disciplines, but the potential benefits for patients and healthcare systems are immense.
Challenges And Limitations In Current AlphaFold Models
AlphaFold, a groundbreaking artificial intelligence system developed by DeepMind, has revolutionized the field of structural biology by predicting protein structures with remarkable accuracy. However, despite its transformative potential, the current AlphaFold models face several challenges and limitations that must be addressed to fully realize their capabilities. Understanding these challenges is crucial for guiding future research and development in this promising area.
One of the primary challenges facing AlphaFold is its reliance on high-quality sequence data. The accuracy of AlphaFold’s predictions is heavily dependent on the availability of comprehensive and accurate protein sequence information. In many cases, especially for less-studied organisms or novel proteins, such data may be incomplete or entirely absent. This limitation can lead to less reliable predictions, particularly for proteins with few homologous sequences available in existing databases. Consequently, expanding and refining protein sequence databases is essential to enhance the predictive power of AlphaFold models.
Moreover, while AlphaFold excels at predicting the static structures of proteins, it struggles with capturing the dynamic nature of these biomolecules. Proteins are not rigid entities; they undergo conformational changes that are critical to their function. AlphaFold’s current models do not adequately account for these dynamic aspects, which can limit their utility in understanding protein function and interactions. Addressing this limitation requires integrating dynamic modeling techniques and experimental data to provide a more comprehensive view of protein behavior.
Another significant limitation is the challenge of predicting protein-protein interactions and complex formation. While AlphaFold has demonstrated success in predicting individual protein structures, the accurate modeling of multi-protein complexes remains a formidable task. The interactions between proteins are often governed by subtle and transient forces that are difficult to capture with current computational models. Enhancing AlphaFold’s ability to predict these interactions will be crucial for advancing our understanding of cellular processes and developing new therapeutic strategies.
Furthermore, the computational demands of AlphaFold present another hurdle. The process of predicting protein structures using AlphaFold requires substantial computational resources, which can be a barrier for many research institutions and laboratories with limited access to high-performance computing facilities. Reducing the computational cost and improving the efficiency of AlphaFold models will be vital for democratizing access to this technology and enabling broader scientific exploration.
In addition to these technical challenges, there are also broader implications related to the interpretation and application of AlphaFold’s predictions. The high accuracy of AlphaFold’s models can sometimes lead to overconfidence in their predictions, potentially overshadowing the need for experimental validation. It is essential to maintain a balanced approach that combines computational predictions with empirical data to ensure the reliability and applicability of the results.
In conclusion, while AlphaFold represents a significant leap forward in protein structure prediction, addressing its current challenges and limitations is crucial for unlocking its full potential. By improving data quality, incorporating dynamic modeling, enhancing interaction predictions, reducing computational demands, and ensuring rigorous validation, the scientific community can pave the way for more comprehensive and impactful applications of AlphaFold technology. As researchers continue to refine and expand upon these models, the future of AlphaFold holds great promise for advancing our understanding of biology and transforming fields such as drug discovery and personalized medicine.
Future Collaborations Between AI And Biochemistry
The intersection of artificial intelligence and biochemistry has been a fertile ground for innovation, with AlphaFold technology standing as a testament to the potential of such collaborations. Developed by DeepMind, AlphaFold has revolutionized the field of protein structure prediction, offering unprecedented accuracy and speed. As we look to the future, the potential for further collaboration between AI and biochemistry is vast, promising to unlock new frontiers in scientific research and practical applications.
AlphaFold’s success in predicting protein structures with remarkable precision has already begun to transform the landscape of molecular biology. This breakthrough has not only accelerated research but also opened up new avenues for drug discovery and development. By providing detailed insights into protein folding, AlphaFold enables researchers to better understand the mechanisms of diseases at a molecular level, paving the way for the design of more effective therapeutics. As we move forward, the integration of AI technologies like AlphaFold with biochemistry will likely become more sophisticated, leading to even more significant advancements.
One of the most promising areas for future collaboration is the development of personalized medicine. By leveraging AlphaFold’s capabilities, researchers can gain a deeper understanding of individual genetic variations and their impact on protein structures. This knowledge can be used to tailor treatments to the specific needs of patients, enhancing the efficacy of interventions and minimizing adverse effects. As AI continues to evolve, its ability to process vast amounts of data and identify patterns will be instrumental in advancing personalized medicine, making it a cornerstone of future healthcare.
Moreover, the synergy between AI and biochemistry is expected to extend beyond human health. Environmental science stands to benefit significantly from these collaborations, particularly in the realm of enzyme engineering. Enzymes play a crucial role in breaking down pollutants and facilitating sustainable industrial processes. By utilizing AlphaFold to predict enzyme structures and functions, scientists can design more efficient enzymes for environmental applications, contributing to efforts in combating climate change and promoting sustainability.
In addition to these applications, the future of AlphaFold technology will likely involve greater collaboration with other AI systems and biotechnological tools. For instance, integrating AlphaFold with CRISPR technology could enhance gene-editing capabilities, allowing for more precise modifications at the molecular level. This could lead to breakthroughs in genetic research and therapy, offering new solutions to previously intractable problems.
Furthermore, as AI continues to advance, the potential for interdisciplinary collaboration will expand. The integration of AlphaFold with other fields such as computational chemistry, systems biology, and synthetic biology could lead to the development of novel materials and bioengineered organisms. These innovations could have far-reaching implications, from creating new materials with unique properties to developing biofuels and other sustainable resources.
In conclusion, the future of AlphaFold technology and its collaboration with biochemistry holds immense promise. As AI continues to evolve and integrate with various scientific disciplines, the potential for groundbreaking discoveries and applications will only grow. By fostering these collaborations, we can look forward to a future where AI and biochemistry work hand in hand to address some of the most pressing challenges facing humanity, from improving healthcare to ensuring environmental sustainability. The journey ahead is filled with possibilities, and the continued partnership between AI and biochemistry will undoubtedly play a pivotal role in shaping the future of science and technology.
Ethical Considerations In The Use Of AlphaFold Technology
The advent of AlphaFold technology has revolutionized the field of structural biology by providing unprecedented insights into protein folding, a process crucial for understanding biological functions and disease mechanisms. As this technology continues to evolve, it is imperative to consider the ethical implications associated with its use. The potential applications of AlphaFold are vast, ranging from drug discovery to synthetic biology, and while these advancements hold promise for significant scientific breakthroughs, they also raise important ethical questions that must be addressed.
One of the primary ethical considerations is the potential for misuse of AlphaFold technology. The ability to predict protein structures with high accuracy could be exploited for nefarious purposes, such as the development of biological weapons. This concern necessitates the establishment of stringent regulatory frameworks to ensure that the technology is used responsibly and for the benefit of society. Moreover, the open-access nature of AlphaFold’s database, while promoting scientific collaboration and innovation, also poses risks if the information is accessed by individuals or groups with malicious intent. Therefore, balancing transparency with security is a critical challenge that must be navigated carefully.
In addition to concerns about misuse, there are ethical considerations related to intellectual property and data ownership. The democratization of protein structure data through AlphaFold has sparked debates about who owns the rights to this information and how it should be shared. Researchers and institutions that have invested significant resources in experimental methods to determine protein structures may feel disadvantaged by the rapid dissemination of computational predictions. Consequently, it is essential to develop fair policies that recognize and reward the contributions of all stakeholders involved in the generation and application of protein structure data.
Furthermore, the integration of AlphaFold technology into drug discovery processes raises ethical questions about access to resulting therapies. While AlphaFold has the potential to accelerate the development of new drugs, there is a risk that these advancements may primarily benefit those in high-income countries, exacerbating existing health disparities. Ensuring equitable access to the fruits of AlphaFold-driven research is a moral imperative that requires international cooperation and policy interventions to prevent the widening of the global health gap.
Another ethical dimension to consider is the impact of AlphaFold on the scientific workforce. As computational methods become more prevalent, there may be a shift in the skills required for careers in structural biology. This transition could lead to job displacement for those whose expertise lies in traditional experimental techniques. To address this, it is crucial to invest in education and training programs that equip scientists with the skills needed to thrive in a landscape increasingly dominated by artificial intelligence and machine learning technologies.
Finally, the use of AlphaFold technology prompts reflection on the broader implications of artificial intelligence in scientific research. As AI systems become more sophisticated, there is a risk of over-reliance on computational predictions, potentially at the expense of experimental validation and critical thinking. It is essential to maintain a balance between embracing technological advancements and preserving the rigor and integrity of scientific inquiry.
In conclusion, while AlphaFold technology offers transformative potential for understanding and manipulating biological systems, it also presents a complex array of ethical challenges. Addressing these issues requires a collaborative effort among scientists, ethicists, policymakers, and the public to ensure that the technology is harnessed in a manner that is safe, equitable, and aligned with societal values. As we look to the future, it is crucial to remain vigilant and proactive in navigating the ethical landscape of this groundbreaking technology.
Q&A
1. **What is AlphaFold?**
AlphaFold is an artificial intelligence program developed by DeepMind that predicts protein structures with high accuracy, significantly advancing the field of structural biology.
2. **How does AlphaFold impact scientific research?**
AlphaFold accelerates scientific research by providing accurate protein structure predictions, which are crucial for understanding biological processes and developing new therapeutics.
3. **What are potential future applications of AlphaFold technology?**
Future applications include drug discovery, personalized medicine, understanding disease mechanisms, and synthetic biology, where accurate protein modeling is essential.
4. **How might AlphaFold evolve in the future?**
AlphaFold may evolve to predict more complex protein interactions, integrate with other biological data, and improve its accuracy and speed, making it more accessible for various research fields.
5. **What challenges does AlphaFold face moving forward?**
Challenges include handling the vast diversity of protein structures, integrating with experimental data, and ensuring predictions are reliable for all types of proteins and conditions.
6. **How could AlphaFold influence biotechnology industries?**
AlphaFold could revolutionize biotechnology by streamlining the design of enzymes, optimizing metabolic pathways, and enhancing the development of novel biomaterials and therapeutics.AlphaFold technology, developed by DeepMind, represents a significant breakthrough in computational biology by accurately predicting protein structures. As we look to the future, AlphaFold’s potential applications are vast and transformative. It promises to accelerate drug discovery, enhance our understanding of diseases, and facilitate the development of novel therapeutics. Moreover, its open-access model democratizes scientific research, enabling global collaboration and innovation. However, challenges remain, including the need for improved accuracy in complex protein interactions and integration with other biological data. Overall, AlphaFold is poised to revolutionize the field of structural biology, offering unprecedented insights into the molecular mechanisms of life.
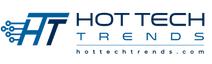