AlphaFold 3 Unveils the Blueprint of Life’s Molecular Interactions
In a groundbreaking advancement at the intersection of artificial intelligence and molecular biology, AlphaFold 3 has emerged as a pivotal tool in decoding the intricate web of life’s molecular interactions. Building upon the revolutionary success of its predecessors, AlphaFold 3 extends its capabilities beyond protein structure prediction to offer unprecedented insights into the dynamic interplay of biomolecules. This latest iteration harnesses cutting-edge machine learning algorithms to map out the complex networks of interactions that underpin biological processes, providing researchers with a comprehensive blueprint of cellular machinery. By elucidating these molecular interactions with remarkable accuracy, AlphaFold 3 is poised to transform our understanding of biological systems, paving the way for novel therapeutic strategies and innovations in biotechnology.
Understanding AlphaFold 3: Revolutionizing Molecular Biology
AlphaFold 3, the latest iteration of the groundbreaking artificial intelligence system developed by DeepMind, is poised to revolutionize the field of molecular biology by providing unprecedented insights into the intricate world of protein structures and their interactions. Building upon the success of its predecessors, AlphaFold 3 offers a more refined and comprehensive understanding of the molecular interactions that underpin life’s biological processes. This advancement is not merely an incremental improvement but a transformative leap that holds the potential to reshape our approach to biological research and drug discovery.
At the core of AlphaFold 3’s innovation is its ability to predict protein structures with remarkable accuracy. Proteins, the workhorses of the cell, are responsible for a myriad of functions, from catalyzing biochemical reactions to providing structural support. Understanding their three-dimensional structures is crucial, as it allows scientists to decipher how proteins interact with each other and with other molecules. AlphaFold 3 employs sophisticated machine learning algorithms to predict these structures based on amino acid sequences, a task that has traditionally been labor-intensive and time-consuming. By automating this process, AlphaFold 3 accelerates research timelines and opens new avenues for exploration.
Moreover, AlphaFold 3 extends its capabilities beyond static protein structures to encompass dynamic molecular interactions. Proteins rarely function in isolation; they interact with other proteins, nucleic acids, and small molecules to carry out their roles. These interactions are often transient and complex, making them challenging to study using conventional methods. AlphaFold 3 addresses this challenge by simulating the dynamic nature of these interactions, providing a more holistic view of cellular processes. This ability to model protein interactions in a dynamic context is a significant advancement, as it allows researchers to explore how proteins change shape and function in response to different stimuli.
In addition to its technical prowess, AlphaFold 3 is democratizing access to structural biology. Traditionally, determining protein structures required access to expensive equipment and specialized expertise, limiting the scope of research to well-funded laboratories. AlphaFold 3, however, is accessible to a broader scientific community, enabling researchers from diverse backgrounds to engage in structural biology research. This democratization fosters collaboration and innovation, as scientists can now explore previously inaccessible areas of study, leading to a more comprehensive understanding of biological systems.
Furthermore, the implications of AlphaFold 3 extend beyond basic research. In the realm of drug discovery, understanding protein structures and interactions is crucial for identifying potential drug targets and designing effective therapeutics. AlphaFold 3’s ability to predict how proteins interact with small molecules can streamline the drug development process, reducing costs and accelerating the timeline for bringing new treatments to market. This capability is particularly relevant in the context of emerging diseases, where rapid response is essential.
In conclusion, AlphaFold 3 represents a monumental advancement in the field of molecular biology, offering a deeper understanding of the molecular interactions that govern life. By accurately predicting protein structures and simulating dynamic interactions, it provides researchers with powerful tools to explore the complexities of biological systems. As AlphaFold 3 continues to evolve, it promises to unlock new frontiers in scientific discovery, paving the way for innovative solutions to some of the most pressing challenges in medicine and biology. Through its transformative impact, AlphaFold 3 is not only enhancing our understanding of life’s molecular blueprint but also shaping the future of scientific research.
AlphaFold 3’s Impact on Drug Discovery and Development
AlphaFold 3, the latest iteration of the groundbreaking protein structure prediction tool developed by DeepMind, is poised to revolutionize the field of drug discovery and development. Building upon the success of its predecessors, AlphaFold 3 offers unprecedented accuracy in predicting protein structures, thereby providing researchers with a more detailed understanding of molecular interactions. This advancement is particularly significant in the realm of drug discovery, where understanding the precise structure of proteins is crucial for designing effective therapeutics.
The process of drug discovery traditionally involves a lengthy and costly trial-and-error approach, often taking years to identify viable drug candidates. However, AlphaFold 3’s ability to predict protein structures with remarkable precision accelerates this process by enabling researchers to visualize the three-dimensional shapes of proteins and their potential binding sites. This capability allows scientists to identify promising drug targets more efficiently, thereby reducing the time and resources required to develop new medications.
Moreover, AlphaFold 3’s impact extends beyond merely identifying drug targets. By providing insights into the dynamic nature of protein interactions, it facilitates the design of molecules that can modulate these interactions in a desired manner. This is particularly beneficial in the development of drugs for complex diseases, such as cancer and neurodegenerative disorders, where the intricate interplay of multiple proteins often dictates disease progression. With AlphaFold 3, researchers can now model these interactions with greater accuracy, leading to the development of more targeted and effective therapies.
In addition to enhancing the drug discovery process, AlphaFold 3 also holds promise for improving the safety and efficacy of new drugs. By predicting how a drug molecule will interact with its target protein, researchers can anticipate potential side effects and modify the drug’s structure accordingly. This proactive approach not only increases the likelihood of clinical success but also minimizes the risk of adverse reactions, ultimately benefiting patients.
Furthermore, AlphaFold 3’s contributions to drug development are not limited to small molecule drugs. The tool is equally valuable in the field of biologics, where understanding protein-protein interactions is essential for designing therapeutic antibodies and other protein-based treatments. By accurately predicting the structures of these complex molecules, AlphaFold 3 enables the rational design of biologics with improved specificity and potency.
The implications of AlphaFold 3’s advancements are far-reaching, extending beyond the pharmaceutical industry to impact various areas of biomedical research. For instance, the tool’s ability to predict protein structures can aid in the study of genetic diseases by revealing how mutations alter protein function. This knowledge can inform the development of gene therapies and other precision medicine approaches, ultimately leading to more personalized and effective treatments.
In conclusion, AlphaFold 3 represents a significant leap forward in our ability to understand and manipulate the molecular underpinnings of life. Its impact on drug discovery and development is profound, offering the potential to streamline the process, enhance drug safety and efficacy, and pave the way for innovative therapies. As researchers continue to harness the power of AlphaFold 3, the future of medicine looks increasingly promising, with the potential to transform the way we approach the treatment of diseases and improve health outcomes worldwide.
The Role of AlphaFold 3 in Unraveling Protein Structures
AlphaFold 3 represents a significant leap forward in the field of computational biology, building upon the groundbreaking achievements of its predecessors to further unravel the complexities of protein structures. Proteins, the workhorses of biological systems, are composed of long chains of amino acids that fold into intricate three-dimensional shapes. These shapes determine the proteins’ functions and interactions within living organisms. Understanding these structures is crucial for advancements in medicine, biotechnology, and fundamental biological research. However, the experimental determination of protein structures, typically through techniques like X-ray crystallography or cryo-electron microscopy, is often time-consuming and resource-intensive. This is where AlphaFold 3 comes into play, offering a transformative approach to predicting protein structures with remarkable accuracy.
AlphaFold 3 builds on the success of AlphaFold 2, which astounded the scientific community by achieving unprecedented levels of precision in protein structure prediction. The latest iteration incorporates advanced machine learning algorithms and a more extensive database of known protein structures, enabling it to predict the folding patterns of proteins with even greater accuracy and speed. This advancement is not merely incremental; it represents a paradigm shift in how scientists approach the study of proteins. By providing detailed insights into the three-dimensional configurations of proteins, AlphaFold 3 facilitates a deeper understanding of their functions and interactions at the molecular level.
One of the most significant contributions of AlphaFold 3 is its ability to predict protein-protein interactions, which are fundamental to virtually all biological processes. Proteins rarely act in isolation; instead, they interact with other proteins to form complex networks that drive cellular functions. Understanding these interactions is essential for elucidating the mechanisms of diseases and developing targeted therapies. AlphaFold 3’s predictive capabilities allow researchers to map these interactions with unprecedented clarity, offering new avenues for drug discovery and the design of novel therapeutics. By simulating how proteins interact with each other, scientists can identify potential binding sites for drugs, paving the way for more effective treatments for a wide range of diseases.
Moreover, AlphaFold 3’s impact extends beyond human health. In agriculture, for instance, understanding protein structures can lead to the development of crops that are more resistant to diseases and environmental stresses. In environmental science, it can aid in the design of enzymes that break down pollutants, contributing to more sustainable practices. The versatility of AlphaFold 3’s applications underscores its potential to drive innovation across multiple fields.
Despite its remarkable capabilities, AlphaFold 3 is not without limitations. The accuracy of its predictions can vary depending on the complexity of the protein and the availability of related structural data. Additionally, while it excels at predicting static structures, the dynamic nature of proteins in living organisms presents an ongoing challenge. Proteins often undergo conformational changes that are critical to their function, and capturing these dynamic processes remains a frontier for future research.
In conclusion, AlphaFold 3 stands as a monumental achievement in the quest to decode the molecular underpinnings of life. By providing detailed insights into protein structures and interactions, it empowers scientists to explore new frontiers in biology, medicine, and beyond. As researchers continue to refine and expand upon this technology, the potential for AlphaFold 3 to revolutionize our understanding of the biological world is immense, promising a future where the mysteries of life at the molecular level are increasingly within our grasp.
How AlphaFold 3 Enhances Our Knowledge of Cellular Processes
AlphaFold 3, the latest iteration of the groundbreaking protein structure prediction tool developed by DeepMind, represents a significant leap forward in our understanding of cellular processes. Building on the success of its predecessors, AlphaFold 3 not only predicts the three-dimensional structures of proteins with remarkable accuracy but also provides insights into the intricate molecular interactions that underpin life’s fundamental processes. This advancement is poised to revolutionize the field of molecular biology, offering unprecedented opportunities to explore the complexities of cellular mechanisms.
To appreciate the impact of AlphaFold 3, it is essential to understand the role of proteins in cellular processes. Proteins are the workhorses of the cell, performing a vast array of functions that are critical to life. They act as enzymes catalyzing biochemical reactions, structural components providing support and shape, and signaling molecules facilitating communication within and between cells. The function of a protein is intimately linked to its three-dimensional structure, which determines how it interacts with other molecules. However, determining protein structures experimentally has been a time-consuming and resource-intensive endeavor, often taking years to complete.
AlphaFold 3 addresses this challenge by leveraging advanced machine learning techniques to predict protein structures with unprecedented speed and accuracy. By analyzing vast amounts of data from known protein structures, AlphaFold 3 has been trained to recognize patterns and relationships that govern protein folding. This capability allows researchers to predict the structures of proteins that have not yet been experimentally determined, thereby accelerating the pace of discovery in molecular biology.
Moreover, AlphaFold 3 goes beyond structure prediction by providing insights into protein-protein interactions, which are crucial for understanding cellular processes. Proteins rarely act in isolation; instead, they interact with other proteins and molecules to form complex networks that drive cellular functions. By accurately predicting how proteins interact with one another, AlphaFold 3 enables researchers to map these networks with greater precision. This knowledge is invaluable for elucidating the molecular basis of diseases, as many pathological conditions arise from aberrant protein interactions.
In addition to enhancing our understanding of protein interactions, AlphaFold 3 also facilitates the study of dynamic processes within cells. Proteins are not static entities; they undergo conformational changes that are essential for their function. AlphaFold 3’s ability to predict these dynamic changes provides insights into how proteins transition between different functional states. This understanding is crucial for unraveling the mechanisms of complex cellular processes such as signal transduction, where proteins relay information through a series of conformational changes.
Furthermore, the implications of AlphaFold 3 extend beyond basic research. In the realm of drug discovery, the ability to predict protein structures and interactions with high accuracy opens new avenues for designing targeted therapeutics. By understanding the precise molecular interactions involved in disease pathways, researchers can develop drugs that specifically modulate these interactions, leading to more effective and less toxic treatments.
In conclusion, AlphaFold 3 represents a transformative advancement in our understanding of cellular processes. By providing accurate predictions of protein structures and interactions, it offers unprecedented insights into the molecular underpinnings of life. This knowledge not only enhances our understanding of fundamental biological processes but also holds promise for advancing medical research and drug discovery. As researchers continue to explore the capabilities of AlphaFold 3, it is clear that this tool will play a pivotal role in shaping the future of molecular biology and medicine.
AlphaFold 3: Bridging the Gap Between Genomics and Proteomics
AlphaFold 3 represents a significant leap forward in the field of bioinformatics, bridging the gap between genomics and proteomics by providing unprecedented insights into the molecular interactions that underpin life. Building on the groundbreaking success of its predecessors, AlphaFold 3 extends the capabilities of protein structure prediction, offering a more comprehensive understanding of how proteins interact within the complex networks of biological systems. This advancement is not merely an incremental improvement but a transformative tool that enhances our ability to decode the intricate language of life at a molecular level.
The journey from genomics to proteomics has always been fraught with challenges, primarily due to the complexity of protein folding and the dynamic nature of protein interactions. While genomics provides the sequence of nucleotides in DNA, it is proteomics that reveals how these sequences translate into functional proteins, the workhorses of the cell. AlphaFold 3 addresses this challenge by accurately predicting the three-dimensional structures of proteins, thereby elucidating how they interact with each other and with other biomolecules. This capability is crucial because the function of a protein is largely determined by its shape and the way it interacts with other molecules.
Transitioning from the static view of individual protein structures, AlphaFold 3 delves into the dynamic realm of protein-protein interactions. It leverages advanced machine learning algorithms to predict not only the structure of individual proteins but also how they assemble into larger complexes. This is a critical development, as many biological processes are governed by the formation and dissolution of these complexes. By providing insights into these interactions, AlphaFold 3 enables researchers to better understand cellular processes such as signal transduction, metabolic pathways, and immune responses.
Moreover, AlphaFold 3’s ability to predict protein interactions has profound implications for drug discovery and development. Traditional methods of drug discovery often involve laborious and time-consuming experimental techniques to determine how potential drug molecules interact with their target proteins. AlphaFold 3 streamlines this process by predicting these interactions computationally, thus accelerating the identification of promising drug candidates. This not only reduces the time and cost associated with drug development but also opens up new avenues for targeting diseases that were previously considered undruggable.
In addition to its applications in drug discovery, AlphaFold 3 holds promise for advancing our understanding of diseases at a molecular level. Many diseases, including cancer and neurodegenerative disorders, are characterized by aberrant protein interactions. By providing a detailed map of these interactions, AlphaFold 3 can help identify the molecular underpinnings of such diseases, paving the way for the development of targeted therapies. Furthermore, its predictive capabilities can be harnessed to explore the effects of genetic mutations on protein structure and function, offering insights into the mechanisms of genetic disorders.
In conclusion, AlphaFold 3 stands as a monumental achievement in the quest to bridge the gap between genomics and proteomics. By unveiling the blueprint of life’s molecular interactions, it not only enhances our understanding of fundamental biological processes but also holds the potential to revolutionize fields such as drug discovery and disease research. As we continue to explore the vast landscape of molecular biology, tools like AlphaFold 3 will undoubtedly play a pivotal role in shaping the future of biomedical research and innovation.
Future Prospects of AlphaFold 3 in Personalized Medicine
The advent of AlphaFold 3 marks a significant milestone in the realm of personalized medicine, offering unprecedented insights into the molecular interactions that underpin human biology. Building upon the groundbreaking achievements of its predecessors, AlphaFold 3 has the potential to revolutionize the way we understand and treat diseases at an individual level. As we delve into the future prospects of this remarkable technology, it becomes evident that its applications could transform personalized medicine, tailoring treatments to the unique genetic makeup of each patient.
To begin with, AlphaFold 3’s ability to predict protein structures with remarkable accuracy opens new avenues for drug discovery and development. Proteins are the workhorses of the cell, and their interactions are fundamental to virtually all biological processes. By providing detailed models of protein structures, AlphaFold 3 enables researchers to identify potential drug targets with greater precision. This capability is particularly crucial in the context of personalized medicine, where understanding the specific molecular interactions in a patient’s body can lead to the development of targeted therapies. Consequently, this could result in more effective treatments with fewer side effects, as drugs can be designed to interact with the unique protein configurations present in an individual’s cells.
Moreover, the integration of AlphaFold 3 into clinical settings could enhance diagnostic accuracy and prognostic assessments. By analyzing the structural variations in proteins that arise from genetic mutations, healthcare professionals can gain deeper insights into the molecular basis of diseases. This knowledge can facilitate the early detection of conditions that might otherwise remain undiagnosed until they reach advanced stages. Furthermore, understanding the structural implications of genetic mutations can aid in predicting disease progression and patient outcomes, allowing for more informed decision-making in treatment planning.
In addition to its diagnostic and therapeutic potential, AlphaFold 3 holds promise for advancing our understanding of complex diseases with multifactorial origins. Conditions such as cancer, neurodegenerative disorders, and autoimmune diseases often involve intricate networks of protein interactions. By elucidating these networks, AlphaFold 3 can help unravel the underlying mechanisms driving disease pathogenesis. This, in turn, could lead to the identification of novel therapeutic targets and the development of innovative treatment strategies that address the root causes of these conditions.
Furthermore, the application of AlphaFold 3 in personalized medicine extends beyond individual patients to encompass population-level health insights. By analyzing protein structures across diverse genetic backgrounds, researchers can identify patterns and variations that contribute to disease susceptibility and resistance. This information can inform public health strategies and guide the development of preventive measures tailored to specific populations. As a result, AlphaFold 3 has the potential to not only improve individual patient outcomes but also enhance the overall health and well-being of communities.
In conclusion, the future prospects of AlphaFold 3 in personalized medicine are both promising and transformative. Its ability to accurately predict protein structures and interactions paves the way for more precise diagnostics, targeted therapies, and a deeper understanding of complex diseases. As this technology continues to evolve, it holds the potential to reshape the landscape of healthcare, ushering in an era where treatments are tailored to the unique molecular blueprint of each individual. Through its integration into clinical practice and research, AlphaFold 3 stands poised to unlock new frontiers in personalized medicine, ultimately improving patient care and outcomes on a global scale.
Q&A
1. **What is AlphaFold 3?**
AlphaFold 3 is the latest iteration of the AlphaFold series, a deep learning model developed by DeepMind to predict protein structures and their molecular interactions with high accuracy.
2. **What advancements does AlphaFold 3 offer over its predecessors?**
AlphaFold 3 enhances the prediction of protein-protein interactions and provides more detailed insights into the dynamic nature of protein structures, improving upon the static predictions of previous versions.
3. **How does AlphaFold 3 impact scientific research?**
AlphaFold 3 accelerates research in fields like drug discovery, molecular biology, and bioengineering by providing precise models of protein interactions, which are crucial for understanding biological processes and designing therapeutics.
4. **What are the potential applications of AlphaFold 3?**
Potential applications include drug design, understanding disease mechanisms, synthetic biology, and personalized medicine, where accurate protein interaction models can lead to breakthroughs in treatment and innovation.
5. **How does AlphaFold 3 achieve its predictions?**
AlphaFold 3 uses advanced machine learning techniques, leveraging vast datasets of known protein structures and interactions to train its models, allowing it to predict unknown structures with high fidelity.
6. **What challenges remain for AlphaFold 3?**
Despite its advancements, challenges include predicting the effects of mutations on protein interactions, modeling multi-protein complexes in diverse environments, and integrating its predictions with experimental data for validation.AlphaFold 3 represents a significant advancement in computational biology, offering unprecedented insights into the molecular interactions that underpin life. By accurately predicting protein structures and their interactions, AlphaFold 3 enhances our understanding of biological processes and accelerates research in drug discovery, disease mechanisms, and synthetic biology. This breakthrough not only deepens our comprehension of the molecular blueprint of life but also opens new avenues for scientific exploration and innovation, potentially transforming the fields of medicine and biotechnology.
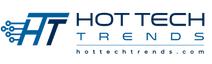