FermiNet is a groundbreaking approach in the field of quantum physics and chemistry, leveraging the power of neural networks to solve complex many-electron systems from first principles. Developed by researchers at DeepMind, FermiNet represents a significant advancement in computational methods, offering a novel way to approximate the wave functions of quantum systems. By utilizing deep learning techniques, FermiNet can efficiently handle the intricate interactions between electrons, providing highly accurate solutions to the Schrödinger equation. This innovative method holds the potential to transform our understanding of quantum mechanics and its applications in chemistry, enabling more precise simulations of molecular structures and reactions, and paving the way for new discoveries in material science and drug design.
Introduction To FermiNet: Revolutionizing Quantum Simulations
FermiNet represents a groundbreaking advancement in the field of quantum simulations, offering a novel approach to understanding the complex interactions that govern quantum physics and chemistry. Developed by researchers at DeepMind, FermiNet is a neural network architecture specifically designed to tackle the many-body problem, a fundamental challenge in quantum mechanics that involves predicting the behavior of systems with multiple interacting particles. This innovative tool has the potential to revolutionize the way scientists simulate quantum systems, providing insights that were previously unattainable with traditional computational methods.
At the heart of FermiNet’s design is its ability to approximate the wave function of a quantum system. The wave function is a mathematical description that encapsulates all the information about a system’s quantum state. However, for systems with many interacting particles, the wave function becomes exceedingly complex, making it difficult to compute accurately. Traditional methods, such as the Hartree-Fock approximation or density functional theory, often rely on simplifying assumptions that can limit their accuracy. In contrast, FermiNet leverages the power of deep learning to model the wave function more precisely, capturing the intricate correlations between particles without resorting to oversimplifications.
One of the key features of FermiNet is its use of antisymmetry, a fundamental property of fermions, which are particles that make up matter, such as electrons. Fermions obey the Pauli exclusion principle, meaning that no two fermions can occupy the same quantum state simultaneously. FermiNet incorporates this principle directly into its architecture, ensuring that the wave function it generates respects the antisymmetry required by quantum mechanics. This built-in antisymmetry allows FermiNet to more accurately model systems of fermions, setting it apart from other neural network approaches that may struggle with this aspect.
Moreover, FermiNet’s architecture is designed to be highly flexible, enabling it to adapt to a wide range of quantum systems. It can be applied to both small molecules and larger, more complex systems, making it a versatile tool for researchers in quantum chemistry and condensed matter physics. By providing a more accurate representation of the wave function, FermiNet allows scientists to explore the properties of materials and molecules with unprecedented precision. This capability opens up new possibilities for discovering novel materials and understanding chemical reactions at a fundamental level.
In addition to its technical capabilities, FermiNet also represents a significant step forward in the integration of machine learning with quantum physics. The use of neural networks to model quantum systems is a relatively new approach, and FermiNet’s success demonstrates the potential of this interdisciplinary field. By combining the strengths of machine learning with the principles of quantum mechanics, FermiNet offers a powerful tool for advancing our understanding of the quantum world.
In conclusion, FermiNet is poised to revolutionize the field of quantum simulations by providing a more accurate and flexible method for modeling complex quantum systems. Its ability to capture the intricate correlations between particles without simplifying assumptions marks a significant departure from traditional approaches. As researchers continue to explore the capabilities of FermiNet, it is likely to play a crucial role in advancing our understanding of quantum physics and chemistry, paving the way for new discoveries and innovations in these fields.
FermiNet’s Role In Quantum Chemistry: A Deep Dive
FermiNet, a groundbreaking neural network architecture, has emerged as a pivotal tool in the exploration of quantum physics and chemistry, offering a novel approach to understanding the fundamental principles governing these fields. At its core, FermiNet is designed to address the complex many-electron Schrödinger equation, a central challenge in quantum chemistry that has long eluded precise solutions due to its computational complexity. By leveraging the power of deep learning, FermiNet provides a promising avenue for accurately modeling the behavior of electrons in molecules, thereby enhancing our understanding of chemical interactions and properties.
The significance of FermiNet lies in its ability to approximate the wave function of a quantum system, which is essential for predicting the energy and properties of molecules. Traditional methods, such as the Hartree-Fock and density functional theory, often rely on approximations that can limit their accuracy, particularly for systems with strong electron correlation. In contrast, FermiNet employs a neural network architecture that directly models the wave function, allowing for a more flexible and accurate representation of electron interactions. This approach not only improves the precision of quantum chemical calculations but also expands the range of systems that can be studied, including those with complex electronic structures.
One of the key innovations of FermiNet is its use of antisymmetry, a fundamental property of fermionic wave functions, which ensures that the wave function changes sign when two electrons are exchanged. This characteristic is crucial for accurately modeling the behavior of electrons, as it reflects the Pauli exclusion principle, a cornerstone of quantum mechanics. By incorporating antisymmetry directly into the neural network architecture, FermiNet is able to capture the essential physics of electron interactions, leading to more reliable predictions of molecular properties.
Moreover, FermiNet’s architecture is designed to be highly scalable, making it suitable for studying a wide range of chemical systems, from small molecules to larger, more complex structures. This scalability is achieved through the use of a deep neural network with multiple layers, each of which captures different aspects of the electron correlation. As a result, FermiNet can be trained to accurately model the wave function of a variety of systems, providing insights into their electronic structure and reactivity.
In addition to its applications in quantum chemistry, FermiNet also holds promise for advancing our understanding of quantum physics more broadly. By providing a powerful tool for solving the many-electron Schrödinger equation, FermiNet can be used to explore fundamental questions in quantum mechanics, such as the nature of entanglement and the behavior of quantum systems under different conditions. This potential for cross-disciplinary research highlights the versatility and impact of FermiNet in advancing our understanding of the quantum world.
In conclusion, FermiNet represents a significant advancement in the field of quantum chemistry, offering a novel approach to solving the many-electron Schrödinger equation with unprecedented accuracy. By leveraging the power of deep learning and incorporating fundamental principles of quantum mechanics, FermiNet provides a powerful tool for exploring the complex interactions that govern chemical systems. As research in this area continues to evolve, FermiNet is poised to play a crucial role in advancing our understanding of both quantum chemistry and physics, paving the way for new discoveries and innovations in these fields.
Understanding The Architecture Of FermiNet
FermiNet, a groundbreaking neural network architecture, represents a significant advancement in the field of quantum physics and chemistry. Developed by researchers at DeepMind, FermiNet is designed to solve the electronic Schrödinger equation, a fundamental equation in quantum mechanics that describes how quantum systems evolve over time. This equation is notoriously difficult to solve for systems with more than a few electrons due to the complex interactions between particles. However, FermiNet offers a novel approach by leveraging the power of deep learning to approximate solutions with remarkable accuracy.
At the core of FermiNet’s architecture is its ability to represent the wave function of a quantum system. The wave function is a mathematical description of the quantum state of a system, encapsulating all the information about the system’s particles. Traditional methods for solving the Schrödinger equation, such as the Hartree-Fock method or density functional theory, often rely on approximations that can limit their accuracy. In contrast, FermiNet uses a neural network to directly model the wave function, allowing it to capture the intricate correlations between electrons more effectively.
The architecture of FermiNet is specifically designed to respect the antisymmetry property of fermionic wave functions. Electrons, being fermions, obey the Pauli exclusion principle, which dictates that no two electrons can occupy the same quantum state simultaneously. This requirement leads to wave functions that change sign when two electrons are exchanged. FermiNet incorporates this antisymmetry by constructing its wave function as a product of determinants, a mathematical structure that naturally exhibits the desired property. This approach enables FermiNet to accurately model the behavior of electrons in a way that traditional neural networks cannot.
Moreover, FermiNet’s architecture is highly flexible, allowing it to be applied to a wide range of quantum systems. It can be used to study small molecules, where it has demonstrated state-of-the-art accuracy in predicting ground state energies. Additionally, FermiNet shows promise in exploring larger and more complex systems, potentially offering insights into materials science and condensed matter physics. This versatility is achieved through the use of a multi-layer perceptron, a type of neural network that can approximate complex functions by stacking multiple layers of neurons. Each layer in FermiNet processes information about the positions and spins of electrons, gradually refining the wave function approximation.
Transitioning from traditional methods to FermiNet involves several challenges, particularly in terms of computational resources. Training a neural network to solve the Schrödinger equation requires significant computational power, as the network must learn to accurately represent the high-dimensional wave function. However, advances in hardware and optimization techniques are continually improving the efficiency of these computations, making FermiNet increasingly accessible to researchers.
In conclusion, FermiNet represents a transformative approach to solving the electronic Schrödinger equation, offering unprecedented accuracy and flexibility. By leveraging the power of deep learning, FermiNet provides a new tool for exploring quantum systems from fundamental principles. Its architecture, designed to respect the unique properties of fermionic wave functions, allows it to capture the complex interactions between electrons with remarkable precision. As computational resources continue to advance, FermiNet holds the potential to unlock new insights into the quantum world, paving the way for breakthroughs in quantum physics and chemistry.
Comparing FermiNet With Traditional Quantum Methods
FermiNet, a neural network-based approach developed by DeepMind, represents a significant advancement in the field of quantum physics and chemistry. It offers a novel method for solving the Schrödinger equation, which is fundamental to understanding the behavior of quantum systems. Traditional quantum methods, such as the Hartree-Fock and Density Functional Theory (DFT), have long been the cornerstone of computational quantum chemistry. However, these methods often involve approximations that can limit their accuracy and applicability, particularly for complex systems. In contrast, FermiNet aims to address these limitations by leveraging the power of machine learning to provide more accurate solutions.
To begin with, traditional methods like Hartree-Fock rely on the mean-field approximation, which simplifies the many-body problem by considering each electron to move in an average field created by all other electrons. While this approach reduces computational complexity, it often fails to capture electron correlation effects accurately. DFT, on the other hand, improves upon Hartree-Fock by incorporating electron correlation through exchange-correlation functionals. However, the exact form of these functionals is unknown, and approximations must be made, which can lead to inaccuracies in certain scenarios.
FermiNet, by contrast, does not rely on such approximations. Instead, it uses a neural network architecture specifically designed to respect the antisymmetry and permutation invariance properties of the electronic wave function. This allows FermiNet to model complex quantum systems more accurately by directly learning from the fundamental principles of quantum mechanics. Moreover, FermiNet’s ability to represent the wave function as a neural network enables it to capture intricate electron correlation effects that traditional methods might miss.
Furthermore, the flexibility of FermiNet’s architecture allows it to be applied to a wide range of quantum systems, from small molecules to larger, more complex systems. This adaptability is a significant advantage over traditional methods, which often require different approaches or significant modifications to handle different types of systems. Additionally, FermiNet’s use of machine learning techniques means that it can improve its accuracy as more data becomes available, potentially leading to even more precise solutions over time.
Despite these advantages, it is important to note that FermiNet is not without its challenges. The computational cost of training neural networks can be substantial, and the method requires significant computational resources, particularly for large systems. However, ongoing advancements in computational power and machine learning algorithms are likely to mitigate these challenges in the future.
In conclusion, FermiNet represents a promising new direction in the study of quantum physics and chemistry. By leveraging the power of neural networks, it offers a more accurate and flexible approach to solving the Schrödinger equation compared to traditional methods. While there are still challenges to overcome, the potential benefits of FermiNet in terms of accuracy and applicability make it a compelling alternative to traditional quantum methods. As research in this area continues to evolve, FermiNet may well become an essential tool for scientists seeking to explore the complexities of quantum systems from fundamental principles.
Applications Of FermiNet In Modern Quantum Research
FermiNet, a neural network architecture developed by DeepMind, has emerged as a groundbreaking tool in the realm of quantum physics and chemistry, offering novel approaches to solving complex quantum systems. Its applications in modern quantum research are vast and varied, providing insights that were previously unattainable through traditional methods. At its core, FermiNet is designed to approximate the wave functions of quantum systems, which are fundamental to understanding the behavior of particles at the quantum level. This capability is particularly significant because wave functions are notoriously difficult to compute, especially for systems with many interacting particles.
One of the primary applications of FermiNet is in the field of quantum chemistry, where it is used to predict the electronic structure of molecules. By accurately modeling the wave functions of electrons, FermiNet allows researchers to calculate the energy levels and properties of molecules with unprecedented precision. This has profound implications for the design of new materials and drugs, as understanding the electronic structure is crucial for predicting how molecules will interact with each other. Consequently, FermiNet is paving the way for advancements in material science and pharmaceuticals, where the ability to predict molecular behavior can lead to the development of more efficient catalysts, better energy storage systems, and novel therapeutic compounds.
Moreover, FermiNet’s applications extend beyond quantum chemistry into the broader field of quantum physics. It is particularly useful in studying quantum many-body systems, which are systems composed of a large number of interacting particles. These systems are notoriously challenging to analyze due to the exponential growth of complexity with the number of particles involved. However, FermiNet’s ability to approximate wave functions makes it a powerful tool for exploring these systems. For instance, it can be used to investigate the properties of quantum materials, such as superconductors and topological insulators, which have potential applications in quantum computing and other advanced technologies.
In addition to its applications in quantum chemistry and physics, FermiNet also holds promise for advancing our understanding of fundamental quantum mechanics. By providing a new method for solving the Schrödinger equation, which is the foundational equation of quantum mechanics, FermiNet offers a fresh perspective on the behavior of quantum systems. This could lead to new insights into the nature of quantum entanglement, coherence, and other phenomena that are central to the field of quantum mechanics.
Furthermore, the development of FermiNet represents a significant step forward in the integration of machine learning with quantum research. The use of neural networks to model quantum systems is a relatively new approach, and FermiNet’s success demonstrates the potential of machine learning to revolutionize the way we study and understand quantum phenomena. As researchers continue to refine and expand upon FermiNet’s capabilities, it is likely that we will see even more innovative applications emerge, further bridging the gap between computational techniques and theoretical physics.
In conclusion, FermiNet is a transformative tool in modern quantum research, with applications that span quantum chemistry, physics, and fundamental quantum mechanics. Its ability to approximate wave functions with high accuracy opens up new possibilities for understanding and manipulating quantum systems, ultimately driving progress in a wide range of scientific and technological fields. As the integration of machine learning and quantum research continues to evolve, FermiNet stands as a testament to the power of interdisciplinary approaches in advancing our knowledge of the quantum world.
Future Prospects Of FermiNet In Quantum Computing
FermiNet, a neural network architecture developed by DeepMind, represents a significant advancement in the field of quantum computing, particularly in the simulation of quantum systems. As researchers continue to explore the potential of FermiNet, its future prospects in quantum computing appear promising. This innovative approach leverages the principles of quantum mechanics to address complex problems in physics and chemistry, offering a new perspective on how we understand and manipulate the quantum world.
One of the most compelling aspects of FermiNet is its ability to model the electronic structure of atoms and molecules with remarkable accuracy. Traditional methods, such as the Hartree-Fock approximation and density functional theory, have long been used to approximate the behavior of electrons in quantum systems. However, these methods often fall short when dealing with strongly correlated systems or when high precision is required. FermiNet, by contrast, employs a neural network to directly approximate the wave function of a quantum system, allowing for a more accurate representation of electron interactions. This capability opens up new avenues for research in quantum chemistry, where understanding the electronic structure is crucial for predicting chemical reactions and designing new materials.
Moreover, the potential applications of FermiNet extend beyond quantum chemistry. In the realm of quantum computing, FermiNet could play a pivotal role in the development of quantum algorithms. As quantum computers continue to evolve, there is a growing need for algorithms that can efficiently solve complex quantum problems. FermiNet’s ability to model quantum systems with high precision makes it a valuable tool for testing and refining these algorithms. By providing insights into the behavior of quantum systems, FermiNet can help researchers identify the most promising approaches for harnessing the power of quantum computing.
In addition to its applications in quantum chemistry and computing, FermiNet also holds promise for advancing our understanding of fundamental physics. Quantum mechanics is a notoriously challenging field, with many phenomena that remain poorly understood. FermiNet’s ability to model quantum systems from first principles offers a new way to explore these phenomena, potentially leading to breakthroughs in our understanding of the quantum world. For instance, FermiNet could be used to study exotic states of matter, such as superconductors and topological insulators, which have significant implications for both fundamental physics and practical applications.
As we look to the future, the continued development of FermiNet and similar neural network architectures will likely play a crucial role in the advancement of quantum computing. However, several challenges remain. One of the primary obstacles is the computational cost associated with training neural networks to model complex quantum systems. As researchers work to overcome these challenges, advances in hardware and algorithmic techniques will be essential to fully realize the potential of FermiNet.
In conclusion, FermiNet represents a significant step forward in the field of quantum computing, with promising applications in quantum chemistry, algorithm development, and fundamental physics. As researchers continue to refine this innovative approach, the future prospects of FermiNet in quantum computing appear bright. By providing a more accurate and efficient way to model quantum systems, FermiNet has the potential to transform our understanding of the quantum world and unlock new possibilities for scientific discovery and technological innovation.
Q&A
1. **What is FermiNet?**
FermiNet is a neural network architecture designed to solve the electronic Schrödinger equation for quantum systems, providing a way to calculate the ground state wavefunctions and energies of molecules and atoms from first principles.
2. **Who developed FermiNet?**
FermiNet was developed by researchers at DeepMind, a subsidiary of Alphabet Inc., known for its work in artificial intelligence and machine learning.
3. **What is the primary goal of FermiNet?**
The primary goal of FermiNet is to accurately model quantum systems by directly approximating the wavefunction of electrons, which allows for precise calculations of molecular properties and interactions without relying on approximations used in traditional quantum chemistry methods.
4. **How does FermiNet differ from traditional quantum chemistry methods?**
Unlike traditional methods that often use approximations like density functional theory (DFT), FermiNet uses deep learning to directly approximate the many-electron wavefunction, potentially offering more accurate results for complex quantum systems.
5. **What are the key components of FermiNet’s architecture?**
FermiNet’s architecture includes a neural network that incorporates the antisymmetry of the wavefunction, electron-electron interactions, and the use of permutation-equivariant layers to respect the indistinguishability of electrons.
6. **What are some potential applications of FermiNet?**
Potential applications of FermiNet include drug discovery, materials science, and any field that requires precise quantum mechanical calculations to understand molecular and atomic interactions.FermiNet represents a significant advancement in the application of deep learning to quantum physics and chemistry, offering a novel approach to solving the electronic Schrödinger equation. By leveraging neural networks to approximate wave functions, FermiNet provides a scalable and flexible framework that can handle complex many-electron systems with high accuracy. This method not only enhances our ability to predict molecular properties and behaviors from first principles but also opens new avenues for research in quantum mechanics and computational chemistry. Its success underscores the potential of integrating machine learning techniques with traditional scientific methodologies to address longstanding challenges in understanding the quantum nature of matter.
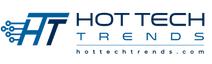