Financial firms are increasingly turning to artificial intelligence (AI) to enhance their operations, improve customer experiences, and gain a competitive edge. However, despite the promising potential of AI, these firms face significant challenges in maximizing its value. The integration of AI technologies into financial services is fraught with complexities, including data privacy concerns, regulatory compliance, and the need for substantial investment in infrastructure and talent. Additionally, the rapid pace of technological advancement requires firms to continuously adapt and innovate, often straining resources and strategic focus. As financial institutions strive to harness the full potential of AI, they must navigate these obstacles to effectively leverage AI-driven insights and solutions, ensuring they not only keep pace with industry trends but also deliver tangible benefits to their stakeholders.
Understanding Data Quality Issues in AI Implementation
In the rapidly evolving landscape of artificial intelligence, financial firms are increasingly seeking to harness the power of AI to gain a competitive edge. However, as these organizations strive to maximize the value of AI, they encounter significant challenges, particularly concerning data quality issues. Understanding and addressing these issues is crucial for the successful implementation of AI technologies in the financial sector.
To begin with, data quality is a foundational element in the development and deployment of AI systems. High-quality data is essential for training accurate and reliable AI models. In the financial industry, where decisions are often based on complex data analysis, the integrity and accuracy of data are paramount. Poor data quality can lead to erroneous predictions, flawed risk assessments, and ultimately, financial losses. Therefore, financial firms must prioritize data quality to ensure that their AI systems function optimally.
One of the primary challenges in maintaining data quality is the sheer volume and variety of data that financial firms handle. With the proliferation of digital transactions, social media interactions, and other data sources, organizations are inundated with vast amounts of information. This data is often unstructured, inconsistent, and incomplete, making it difficult to process and analyze effectively. Consequently, financial firms must invest in robust data management systems and employ advanced data cleaning techniques to ensure that their datasets are accurate and reliable.
Moreover, data quality issues are exacerbated by the presence of legacy systems within many financial institutions. These outdated systems often store data in disparate formats and silos, hindering seamless data integration and analysis. As a result, financial firms face the daunting task of modernizing their IT infrastructure to facilitate better data management. This involves not only upgrading technology but also fostering a culture of data governance, where data quality is continuously monitored and improved.
In addition to technical challenges, financial firms must also navigate regulatory and ethical considerations related to data quality. Regulatory bodies impose stringent requirements on data handling and privacy, necessitating that firms implement robust compliance measures. Ensuring data quality in this context involves not only adhering to legal standards but also maintaining transparency and accountability in data usage. Ethical considerations, such as avoiding bias in AI models, further complicate the data quality landscape. Financial firms must be vigilant in identifying and mitigating biases that may arise from poor-quality data, as these can lead to unfair or discriminatory outcomes.
Furthermore, the dynamic nature of financial markets adds another layer of complexity to data quality issues. Market conditions can change rapidly, rendering historical data less relevant or even obsolete. Financial firms must therefore develop adaptive AI models that can account for these fluctuations and continue to deliver accurate insights. This requires a continuous feedback loop where AI systems are regularly updated with fresh, high-quality data to maintain their effectiveness.
In conclusion, while AI holds immense potential for transforming the financial industry, the challenges associated with data quality cannot be overlooked. Financial firms must adopt a comprehensive approach to data management, encompassing technological upgrades, regulatory compliance, and ethical considerations. By addressing these data quality issues, organizations can unlock the full potential of AI, driving innovation and achieving sustainable growth in an increasingly competitive market. As the financial sector continues to evolve, the ability to effectively manage and leverage high-quality data will be a key determinant of success in AI implementation.
Overcoming Talent Shortages in AI Development
In the rapidly evolving landscape of artificial intelligence, financial firms are increasingly recognizing the transformative potential of AI technologies. However, as these organizations strive to harness AI’s capabilities, they encounter a significant hurdle: a shortage of skilled talent. This talent gap poses a formidable challenge in maximizing the value that AI can bring to the financial sector. To address this issue, firms must adopt strategic approaches that not only attract but also nurture and retain AI talent.
Firstly, it is essential to understand the root causes of the talent shortage in AI development. The demand for AI expertise has surged across various industries, leading to fierce competition for skilled professionals. Financial firms, in particular, face the dual challenge of competing with tech giants and startups that often offer more flexible and innovative work environments. Moreover, the rapid pace of AI advancements means that the required skill sets are continually evolving, making it difficult for educational institutions to keep curricula up-to-date. Consequently, there is a mismatch between the skills that graduates possess and those that are in demand.
To overcome these challenges, financial firms must adopt a multi-faceted approach. One effective strategy is to invest in upskilling and reskilling existing employees. By providing continuous learning opportunities, firms can bridge the skills gap and ensure that their workforce remains competitive. This can be achieved through partnerships with educational institutions, online courses, and in-house training programs. Additionally, fostering a culture of innovation and learning within the organization can motivate employees to pursue further education and skill development.
Furthermore, financial firms should consider diversifying their talent acquisition strategies. Traditional recruitment methods may not suffice in attracting top AI talent. Instead, firms can explore collaborations with universities and research institutions to tap into emerging talent pools. Internship programs and research partnerships can serve as effective pipelines for identifying and nurturing potential candidates. Additionally, leveraging global talent through remote work arrangements can expand the pool of available expertise, allowing firms to access skilled professionals from different geographical locations.
Another critical aspect of overcoming talent shortages is creating an attractive work environment that appeals to AI professionals. Competitive compensation packages are undoubtedly important, but they are not the sole factor in attracting talent. Financial firms should focus on offering challenging and meaningful projects that allow AI professionals to apply their skills creatively. Providing opportunities for career advancement and professional growth can also enhance job satisfaction and retention.
Moreover, fostering a diverse and inclusive workplace can significantly impact talent acquisition and retention. Diverse teams bring varied perspectives and ideas, which can drive innovation and problem-solving. By promoting diversity and inclusion, financial firms can create an environment where AI professionals from different backgrounds feel valued and motivated to contribute their best work.
In conclusion, while the talent shortage in AI development presents a significant challenge for financial firms, it is not insurmountable. By investing in upskilling, diversifying recruitment strategies, and creating an attractive work environment, firms can effectively address this issue. As the financial sector continues to evolve, those organizations that successfully navigate the talent landscape will be better positioned to maximize the value of AI technologies, ultimately gaining a competitive edge in the market. Through strategic efforts and a commitment to fostering talent, financial firms can unlock the full potential of AI and drive innovation in the industry.
Navigating Regulatory Compliance in AI Utilization
As financial firms increasingly turn to artificial intelligence (AI) to enhance their operations, they encounter a complex landscape of regulatory compliance that poses significant challenges. The integration of AI into financial services promises numerous benefits, including improved efficiency, enhanced customer experiences, and more accurate risk assessments. However, the regulatory environment surrounding AI utilization is evolving rapidly, and firms must navigate this terrain carefully to maximize the value of their AI investments.
To begin with, the financial industry is heavily regulated, and the introduction of AI technologies adds a layer of complexity to compliance efforts. Regulators are keenly aware of the potential risks associated with AI, such as algorithmic bias, data privacy concerns, and the lack of transparency in decision-making processes. Consequently, they are developing frameworks and guidelines to ensure that AI applications in finance adhere to ethical standards and do not compromise consumer protection. Financial firms must stay abreast of these regulatory developments to avoid potential pitfalls and ensure that their AI systems are compliant.
Moreover, the global nature of financial markets means that firms often operate across multiple jurisdictions, each with its own set of regulations. This diversity in regulatory requirements can create challenges for firms seeking to implement AI solutions on a global scale. For instance, the European Union’s General Data Protection Regulation (GDPR) imposes stringent data protection and privacy requirements that may differ from those in other regions. As a result, financial firms must adopt a flexible approach to AI deployment, ensuring that their systems can adapt to varying regulatory landscapes while maintaining compliance.
In addition to understanding and adhering to existing regulations, financial firms must also anticipate future regulatory changes. The rapid pace of technological advancement means that regulators are continually reassessing their frameworks to address emerging risks and opportunities. Firms that proactively engage with regulators and participate in industry discussions are better positioned to influence policy development and ensure that their AI strategies align with evolving regulatory expectations. This proactive approach not only helps firms mitigate compliance risks but also enables them to capitalize on new opportunities as they arise.
Furthermore, transparency and explainability are critical components of regulatory compliance in AI utilization. Financial firms must ensure that their AI systems are not only effective but also understandable to regulators, customers, and other stakeholders. This requires the development of robust governance frameworks that include clear documentation of AI models, data sources, and decision-making processes. By fostering transparency, firms can build trust with regulators and customers alike, thereby enhancing their reputation and competitive advantage.
Finally, collaboration and knowledge sharing within the industry can play a vital role in navigating regulatory compliance challenges. Financial firms can benefit from participating in industry consortia, working groups, and forums that focus on AI and regulatory issues. These platforms provide opportunities for firms to share best practices, learn from each other’s experiences, and collectively address common challenges. By fostering a collaborative approach, the industry can work towards developing standardized practices and guidelines that facilitate compliance and promote the responsible use of AI.
In conclusion, while the integration of AI into financial services offers significant potential, it also presents a complex regulatory landscape that firms must navigate carefully. By staying informed of regulatory developments, adopting flexible and transparent AI strategies, and engaging in industry collaboration, financial firms can effectively manage compliance challenges and maximize the value of their AI investments.
Addressing Ethical Concerns in AI Decision-Making
In the rapidly evolving landscape of artificial intelligence, financial firms are increasingly leveraging AI technologies to enhance decision-making processes, optimize operations, and deliver personalized services. However, as these firms strive to maximize the value derived from AI, they encounter significant challenges, particularly in addressing ethical concerns associated with AI decision-making. The integration of AI into financial services necessitates a careful examination of ethical implications to ensure that the deployment of these technologies aligns with societal values and regulatory standards.
One of the primary ethical concerns in AI decision-making is the potential for bias. AI systems, which are trained on historical data, can inadvertently perpetuate existing biases present in the data. This is particularly problematic in the financial sector, where decisions regarding creditworthiness, loan approvals, and risk assessments can significantly impact individuals’ lives. If not addressed, biased AI systems can lead to unfair treatment of certain groups, exacerbating social inequalities. Therefore, financial firms must implement robust mechanisms to identify and mitigate bias in AI algorithms, ensuring that these systems operate fairly and equitably.
Moreover, transparency in AI decision-making is another critical ethical consideration. The complexity of AI models, particularly those based on deep learning, often results in a “black box” phenomenon, where the decision-making process is not easily interpretable. This lack of transparency can undermine trust in AI systems, as stakeholders may be unable to understand or challenge the rationale behind certain decisions. To address this issue, financial firms are increasingly focusing on developing explainable AI models that provide clear and understandable insights into how decisions are made. By enhancing transparency, these firms can foster greater trust and confidence among clients and regulators.
In addition to bias and transparency, the ethical use of data is a significant concern in AI decision-making. Financial firms have access to vast amounts of sensitive data, and the use of this data in AI systems raises questions about privacy and consent. It is imperative for firms to establish stringent data governance frameworks that prioritize the protection of personal information and ensure compliance with data protection regulations. By doing so, they can safeguard individuals’ privacy while harnessing the power of AI to drive innovation and efficiency.
Furthermore, accountability in AI decision-making is essential to address ethical concerns. As AI systems become more autonomous, determining responsibility for decisions made by these systems becomes increasingly complex. Financial firms must establish clear accountability structures that delineate the roles and responsibilities of human and machine agents in the decision-making process. This involves not only defining who is accountable for AI-driven decisions but also ensuring that there are mechanisms in place to rectify any adverse outcomes resulting from these decisions.
In conclusion, while AI offers immense potential for financial firms to enhance their decision-making capabilities, it also presents significant ethical challenges that must be addressed to maximize its value. By focusing on mitigating bias, enhancing transparency, ensuring ethical data use, and establishing accountability, financial firms can navigate the complex ethical landscape of AI decision-making. As these firms continue to integrate AI into their operations, a commitment to ethical principles will be crucial in building trust, maintaining regulatory compliance, and ultimately achieving sustainable success in the digital age.
Integrating AI with Legacy Systems in Financial Firms
The integration of artificial intelligence (AI) into legacy systems within financial firms presents a complex challenge that requires careful navigation. As financial institutions strive to harness the transformative potential of AI, they are often confronted with the intricate task of merging cutting-edge technology with existing, and sometimes outdated, infrastructure. This endeavor is not merely a technical challenge but also a strategic one, as firms must balance innovation with the stability and reliability that legacy systems have historically provided.
To begin with, legacy systems in financial firms are deeply entrenched, having been developed and refined over decades to handle vast amounts of data and transactions securely. These systems are often characterized by their robustness and reliability, qualities that are indispensable in the financial sector. However, they are also typically inflexible and resistant to change, which poses a significant hurdle when attempting to integrate AI technologies. The rigidity of these systems can impede the seamless adoption of AI, which thrives on flexibility and adaptability to process and analyze data in real-time.
Moreover, the integration process is further complicated by the sheer volume and complexity of data that financial firms manage. AI systems require access to large datasets to function effectively, yet legacy systems may not be equipped to handle the data influx that AI demands. This necessitates the development of sophisticated data management strategies to ensure that AI can be effectively integrated without overwhelming existing systems. Financial firms must invest in upgrading their data infrastructure to facilitate the smooth flow of information between AI and legacy systems, ensuring that data is both accessible and secure.
In addition to technical challenges, there are also significant cultural and organizational barriers to consider. The introduction of AI into financial firms often requires a shift in mindset, as employees must adapt to new ways of working and decision-making. This can be a daunting prospect for organizations that have long relied on traditional methods and processes. To overcome this, firms must foster a culture of innovation and continuous learning, encouraging employees to embrace AI as a tool that can enhance, rather than replace, their expertise.
Furthermore, regulatory compliance is a critical consideration in the financial sector, and the integration of AI must be carefully managed to ensure adherence to stringent regulations. Financial firms must work closely with regulators to navigate the complex landscape of compliance, ensuring that AI systems are transparent, accountable, and secure. This requires a proactive approach to governance, with firms implementing robust frameworks to monitor and manage AI systems effectively.
Despite these challenges, the potential benefits of integrating AI with legacy systems are substantial. AI can enhance decision-making, improve operational efficiency, and provide valuable insights that drive innovation and growth. By leveraging AI, financial firms can gain a competitive edge, offering more personalized and efficient services to their clients. However, realizing these benefits requires a strategic approach to integration, with firms investing in the necessary infrastructure, skills, and governance frameworks to support AI adoption.
In conclusion, while the integration of AI with legacy systems in financial firms presents significant challenges, it also offers immense opportunities for those willing to invest in the necessary resources and strategies. By addressing technical, organizational, and regulatory hurdles, financial firms can unlock the full potential of AI, driving innovation and growth in an increasingly competitive landscape.
Measuring ROI and Performance of AI Investments
In the rapidly evolving landscape of financial services, artificial intelligence (AI) has emerged as a transformative force, promising to revolutionize everything from customer service to risk management. However, as financial firms increasingly invest in AI technologies, they face significant challenges in measuring the return on investment (ROI) and performance of these initiatives. Understanding the complexities involved in quantifying AI’s value is crucial for firms aiming to maximize their investments and maintain a competitive edge.
To begin with, one of the primary challenges in measuring AI’s ROI is the intangible nature of many of its benefits. Unlike traditional investments, where returns can be easily quantified in monetary terms, AI often delivers value through enhanced customer experiences, improved decision-making, and increased operational efficiency. These benefits, while substantial, are not always directly reflected in financial statements, making it difficult for firms to assess the true impact of their AI initiatives. Consequently, financial firms must develop new metrics and evaluation frameworks that capture both the tangible and intangible benefits of AI.
Moreover, the performance of AI systems is inherently tied to the quality and quantity of data available. Financial firms, therefore, need to ensure that they have robust data management practices in place to support their AI initiatives. This involves not only collecting and storing vast amounts of data but also ensuring its accuracy, relevance, and timeliness. Without high-quality data, AI systems may produce unreliable or biased results, undermining their effectiveness and, by extension, their perceived value. Thus, investing in data infrastructure and governance is a critical component of maximizing AI’s ROI.
In addition to data challenges, financial firms must also navigate the complexities of integrating AI into existing systems and processes. AI technologies often require significant changes to organizational workflows, necessitating employee training and adjustments to corporate culture. Resistance to change can impede the successful implementation of AI, thereby affecting its performance and ROI. To address this, firms should prioritize change management strategies that foster a culture of innovation and adaptability. By doing so, they can ensure that their workforce is equipped to leverage AI technologies effectively, thereby enhancing the overall value derived from these investments.
Furthermore, the dynamic nature of AI technology presents another layer of complexity in measuring its performance. As AI continues to evolve, financial firms must stay abreast of the latest developments to ensure that their investments remain relevant and effective. This requires ongoing monitoring and evaluation of AI systems to identify areas for improvement and adaptation. By adopting a continuous improvement mindset, firms can optimize their AI investments and ensure that they deliver sustained value over time.
Finally, regulatory considerations also play a crucial role in shaping the ROI and performance of AI investments in the financial sector. As regulators increasingly scrutinize the use of AI, firms must ensure that their systems comply with relevant laws and ethical standards. This involves implementing robust governance frameworks that address issues such as data privacy, algorithmic transparency, and accountability. By proactively managing these regulatory challenges, financial firms can mitigate potential risks and enhance the trust and confidence of their stakeholders.
In conclusion, while AI holds immense potential for transforming the financial services industry, realizing its full value requires a nuanced approach to measuring ROI and performance. By addressing challenges related to data quality, integration, technological evolution, and regulatory compliance, financial firms can unlock the true potential of their AI investments and drive sustainable growth in an increasingly competitive market.
Q&A
1. **Question:** What are some common challenges financial firms face in maximizing AI value?
**Answer:** Financial firms often encounter challenges such as data quality and integration issues, regulatory compliance hurdles, lack of skilled personnel, high implementation costs, and difficulties in aligning AI initiatives with business goals.
2. **Question:** How does data quality impact the effectiveness of AI in financial firms?
**Answer:** Poor data quality can lead to inaccurate AI models, resulting in unreliable predictions and insights. This can undermine decision-making processes and reduce the overall value derived from AI investments.
3. **Question:** Why is regulatory compliance a significant challenge for financial firms using AI?
**Answer:** Financial firms must navigate complex regulatory environments that require transparency, accountability, and fairness in AI applications. Ensuring compliance can be resource-intensive and may limit the scope of AI deployment.
4. **Question:** What role does talent play in the successful implementation of AI in financial firms?
**Answer:** Skilled personnel are crucial for developing, deploying, and maintaining AI systems. A shortage of AI talent can hinder a firm’s ability to effectively leverage AI technologies and extract maximum value.
5. **Question:** How do high implementation costs affect AI adoption in financial firms?
**Answer:** The substantial investment required for AI infrastructure, tools, and talent can be a barrier for many financial firms, especially smaller ones, limiting their ability to adopt and scale AI solutions.
6. **Question:** In what ways can financial firms align AI initiatives with business goals?
**Answer:** Firms can align AI initiatives with business goals by clearly defining objectives, involving stakeholders from various departments, ensuring AI projects address specific business needs, and continuously measuring and adjusting AI strategies to achieve desired outcomes.Financial firms face significant challenges in maximizing the value of AI due to several factors. These include the complexity of integrating AI technologies into existing systems, the need for substantial investment in infrastructure and talent, and the difficulty in managing data quality and privacy concerns. Additionally, regulatory compliance and ethical considerations pose further obstacles. Despite these challenges, firms that successfully navigate these issues can achieve enhanced decision-making, improved customer experiences, and operational efficiencies. However, realizing these benefits requires a strategic approach, focusing on aligning AI initiatives with business goals, fostering a culture of innovation, and ensuring robust governance frameworks.
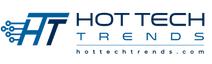