In the rapidly evolving landscape of artificial intelligence, the efficacy of AI systems is increasingly contingent upon the quality of data they are trained on. As organizations strive to harness the power of AI for competitive advantage, a critical question emerges: Is your AI suffering from inadequate data quality? Poor data quality can significantly undermine the performance and reliability of AI models, leading to inaccurate predictions, biased outcomes, and ultimately, flawed decision-making. This issue not only hampers the potential benefits of AI but also poses substantial risks to businesses and their stakeholders. Understanding the implications of data quality and implementing robust data management practices are essential steps in ensuring that AI systems operate at their full potential, delivering accurate and trustworthy results.
Understanding Data Quality: The Foundation of Effective AI
In the rapidly evolving landscape of artificial intelligence, the importance of data quality cannot be overstated. As organizations increasingly rely on AI to drive decision-making, enhance customer experiences, and streamline operations, the foundation upon which these systems are built—data—becomes critically important. Understanding data quality is essential for ensuring that AI systems function effectively and deliver accurate, reliable results. Without high-quality data, even the most sophisticated AI models can falter, leading to erroneous conclusions and potentially costly mistakes.
To begin with, data quality encompasses several dimensions, including accuracy, completeness, consistency, timeliness, and relevance. Accuracy refers to the correctness of the data, ensuring that it reflects real-world conditions without errors. Completeness involves having all necessary data points available, while consistency ensures that data is uniform across different datasets and systems. Timeliness relates to the data being up-to-date, and relevance ensures that the data is pertinent to the task at hand. Each of these dimensions plays a crucial role in the overall quality of data, and deficiencies in any area can significantly impact AI performance.
Transitioning to the implications of poor data quality, it is important to recognize that AI systems are only as good as the data they are trained on. Inadequate data quality can lead to biased models, as AI systems may learn and perpetuate inaccuracies present in the training data. This can result in skewed predictions and decisions that do not align with reality. For instance, if an AI model is trained on incomplete or outdated data, it may fail to recognize emerging trends or changes in patterns, leading to suboptimal outcomes. Moreover, inconsistencies in data can cause AI systems to behave unpredictably, undermining trust and reliability.
Furthermore, the impact of poor data quality extends beyond technical performance. It can also have significant business implications. Organizations may face financial losses due to incorrect predictions or decisions made by AI systems. Additionally, there is a reputational risk associated with deploying AI models that produce biased or inaccurate results. Customers and stakeholders expect AI-driven solutions to be fair and reliable, and any deviation from these expectations can damage an organization’s credibility and trustworthiness.
To mitigate these risks, organizations must prioritize data quality management as a fundamental aspect of their AI strategy. This involves implementing robust data governance frameworks, which include processes for data collection, validation, and cleansing. Regular audits and monitoring of data quality are essential to identify and rectify issues promptly. Moreover, fostering a culture of data literacy within the organization can empower employees to recognize the importance of data quality and contribute to its maintenance.
In conclusion, the foundation of effective AI lies in the quality of the data it is built upon. As AI continues to permeate various aspects of business and society, ensuring high data quality is paramount. By understanding and addressing the dimensions of data quality, organizations can enhance the performance and reliability of their AI systems, ultimately leading to better decision-making and improved outcomes. As we move forward in this data-driven era, prioritizing data quality will be a key differentiator for organizations seeking to harness the full potential of AI.
Common Data Quality Issues That Impact AI Performance
In the rapidly evolving landscape of artificial intelligence, the quality of data used to train and operate AI systems is paramount. As organizations increasingly rely on AI to drive decision-making and automate processes, the integrity of the data feeding these systems becomes a critical factor in their success. Poor data quality can significantly impair AI performance, leading to inaccurate predictions, biased outcomes, and ultimately, a loss of trust in AI solutions. Understanding common data quality issues is essential for mitigating these risks and ensuring that AI systems function optimally.
One prevalent issue is the presence of incomplete data. Incomplete datasets can arise from various sources, such as missing entries in data collection processes or gaps in historical data. When AI models are trained on incomplete data, they may struggle to identify patterns or make accurate predictions, as they lack a comprehensive view of the information needed. This can result in models that are less robust and more prone to errors, particularly when faced with new or unexpected scenarios.
In addition to incompleteness, inconsistency in data is another significant challenge. Inconsistent data can occur when there are discrepancies in data formats, units of measurement, or naming conventions across different datasets. Such inconsistencies can confuse AI algorithms, leading to erroneous interpretations and flawed outputs. For instance, if one dataset records temperature in Celsius while another uses Fahrenheit, an AI model may produce incorrect results unless these differences are reconciled. Ensuring consistency in data formatting and standardization is crucial for maintaining the reliability of AI systems.
Moreover, data accuracy is a fundamental aspect of data quality that directly impacts AI performance. Inaccurate data can stem from human errors during data entry, faulty sensors, or outdated information. When AI models are trained on inaccurate data, they are likely to generate unreliable predictions and decisions. This can have serious consequences, particularly in high-stakes applications such as healthcare or finance, where precision is critical. Regular data validation and verification processes are necessary to identify and rectify inaccuracies, thereby enhancing the overall quality of the data.
Bias in data is another critical issue that can adversely affect AI performance. Bias can be introduced at various stages, from data collection to labeling, and can result in AI models that perpetuate or even exacerbate existing prejudices. For example, if a dataset used to train a facial recognition system predominantly consists of images of individuals from a specific demographic group, the system may perform poorly when identifying individuals from other groups. Addressing bias requires a concerted effort to ensure diverse and representative datasets, as well as ongoing monitoring and adjustment of AI models to mitigate biased outcomes.
Finally, the timeliness of data is an often-overlooked aspect of data quality. In many applications, particularly those involving real-time decision-making, outdated data can lead to suboptimal or even harmful outcomes. AI systems must be fed with up-to-date information to remain relevant and effective. Implementing mechanisms for continuous data updates and real-time data integration can help maintain the timeliness and relevance of the data used by AI systems.
In conclusion, addressing common data quality issues is essential for optimizing AI performance and ensuring reliable outcomes. By focusing on completeness, consistency, accuracy, bias, and timeliness, organizations can enhance the quality of their data and, consequently, the effectiveness of their AI systems. As AI continues to play an increasingly integral role in various sectors, prioritizing data quality will be crucial for harnessing its full potential and building trust in AI-driven solutions.
Strategies for Improving Data Quality in AI Systems
In the rapidly evolving landscape of artificial intelligence, the quality of data used to train AI systems is paramount. Poor data quality can lead to inaccurate predictions, biased outcomes, and ultimately, a lack of trust in AI applications. Therefore, it is crucial to implement strategies that enhance data quality, ensuring that AI systems perform optimally and ethically. One effective strategy is to establish a robust data governance framework. This involves setting clear guidelines and standards for data collection, storage, and processing. By doing so, organizations can ensure that data is consistently accurate, complete, and relevant. Moreover, a well-defined governance framework facilitates accountability and transparency, which are essential for maintaining data integrity.
In addition to governance, data preprocessing is a critical step in improving data quality. This process involves cleaning the data to remove inaccuracies, such as duplicates or missing values, and transforming it into a format suitable for analysis. Techniques such as normalization and standardization can be employed to ensure that data is consistent and comparable across different datasets. Furthermore, data augmentation can be used to enhance the diversity and richness of the dataset, thereby improving the robustness of AI models. By augmenting data, organizations can simulate various scenarios and conditions, which helps in training AI systems to handle real-world complexities more effectively.
Another strategy to consider is the implementation of continuous data quality monitoring. This involves regularly assessing the quality of data through automated tools and techniques. By continuously monitoring data quality, organizations can quickly identify and rectify issues before they impact AI performance. This proactive approach not only ensures the reliability of AI systems but also enhances their adaptability to changing data environments. Additionally, leveraging machine learning algorithms for anomaly detection can further aid in identifying data quality issues that may not be immediately apparent.
Collaboration between data scientists and domain experts is also vital in improving data quality. Domain experts possess the contextual knowledge necessary to interpret data accurately and identify potential biases or errors. By working closely with data scientists, they can provide valuable insights that enhance the quality and relevance of the data used in AI systems. This collaborative approach ensures that AI models are not only technically sound but also contextually appropriate, thereby increasing their effectiveness and trustworthiness.
Moreover, organizations should prioritize the ethical considerations of data usage. This involves ensuring that data is collected and used in compliance with legal and ethical standards, such as data privacy regulations. By adopting ethical data practices, organizations can mitigate the risk of bias and discrimination in AI systems, thereby fostering trust among users and stakeholders. Furthermore, ethical data practices contribute to the development of AI systems that are fair and inclusive, which is essential for their widespread acceptance and adoption.
In conclusion, improving data quality in AI systems is a multifaceted endeavor that requires a combination of governance, preprocessing, monitoring, collaboration, and ethical considerations. By implementing these strategies, organizations can enhance the performance, reliability, and trustworthiness of their AI applications. As AI continues to permeate various aspects of society, ensuring high data quality will be crucial in unlocking its full potential and achieving positive outcomes.
The Role of Data Cleaning in Enhancing AI Accuracy
In the rapidly evolving landscape of artificial intelligence, the accuracy and reliability of AI models are paramount. As organizations increasingly rely on AI to drive decision-making processes, the quality of the data feeding these models becomes a critical factor. Poor data quality can lead to inaccurate predictions, flawed insights, and ultimately, misguided decisions. Therefore, data cleaning emerges as an essential process in enhancing AI accuracy, ensuring that the models are trained on reliable and relevant information.
Data cleaning, often referred to as data cleansing or data scrubbing, involves the detection and correction of errors and inconsistencies in datasets. This process is crucial because raw data, as it is collected from various sources, is often riddled with inaccuracies, missing values, duplicates, and irrelevant information. These imperfections can significantly impair the performance of AI models, leading to skewed results and unreliable outputs. Consequently, data cleaning serves as a foundational step in preparing data for analysis, ensuring that the subsequent AI processes are built on a solid and trustworthy base.
One of the primary benefits of data cleaning is the enhancement of data accuracy. By identifying and rectifying errors, data cleaning ensures that the information fed into AI models is as precise as possible. This accuracy is vital for models that rely on historical data to predict future trends or make recommendations. For instance, in the healthcare sector, where AI is used to predict patient outcomes or recommend treatments, inaccurate data can have serious implications. By cleaning the data, healthcare providers can ensure that their AI systems are making decisions based on the most accurate and up-to-date information available.
Moreover, data cleaning helps in improving data consistency. In many cases, data is collected from multiple sources, each with its own format and standards. This inconsistency can lead to confusion and errors when the data is integrated into a single dataset. Through data cleaning, organizations can standardize the data, ensuring that it is uniform and coherent. This consistency is crucial for AI models that require a harmonized dataset to function effectively. By eliminating discrepancies, data cleaning facilitates a smoother integration process, allowing AI models to operate more efficiently and accurately.
In addition to accuracy and consistency, data cleaning also plays a significant role in enhancing data completeness. Missing values are a common issue in datasets, and they can severely impact the performance of AI models. Data cleaning involves identifying these gaps and employing strategies to address them, such as imputation or interpolation. By filling in missing values, data cleaning ensures that AI models have access to a complete dataset, enabling them to make more informed and reliable predictions.
Furthermore, data cleaning contributes to the reduction of data redundancy. Duplicate records can skew the results of AI models, leading to biased outcomes. By identifying and removing these duplicates, data cleaning ensures that each data point is unique and contributes meaningfully to the analysis. This reduction in redundancy not only improves the efficiency of AI models but also enhances their accuracy by providing a more representative dataset.
In conclusion, the role of data cleaning in enhancing AI accuracy cannot be overstated. By improving data accuracy, consistency, completeness, and reducing redundancy, data cleaning lays the groundwork for reliable and effective AI models. As organizations continue to harness the power of AI, investing in robust data cleaning processes will be essential to ensure that their AI systems deliver accurate and actionable insights.
How Poor Data Quality Can Lead to AI Bias
In the rapidly evolving landscape of artificial intelligence, the quality of data used to train AI models is of paramount importance. Poor data quality can significantly impact the performance and reliability of AI systems, leading to unintended consequences such as bias. As organizations increasingly rely on AI to make critical decisions, understanding the implications of inadequate data quality becomes essential.
To begin with, data quality issues can arise from various sources, including incomplete data, outdated information, and inaccuracies. When AI models are trained on such flawed datasets, they are likely to produce skewed results. For instance, if an AI system is trained on a dataset that lacks diversity, it may fail to accurately represent the broader population. This can lead to biased outcomes, particularly in applications such as hiring, lending, and law enforcement, where fairness and equity are crucial.
Moreover, the presence of historical biases in training data can perpetuate and even amplify existing prejudices. AI systems learn patterns from the data they are fed, and if these patterns reflect societal biases, the AI will likely replicate them. For example, if a dataset used to train a hiring algorithm predominantly features successful candidates from a particular demographic, the AI may inadvertently favor similar profiles in future hiring decisions. This not only undermines the fairness of the AI system but also raises ethical concerns about discrimination and inequality.
In addition to historical biases, data quality issues can also stem from the way data is collected and labeled. Inaccurate labeling or misclassification of data points can lead to erroneous conclusions by AI models. For instance, if an image recognition system is trained on poorly labeled images, it may struggle to correctly identify objects in real-world scenarios. This highlights the importance of meticulous data curation and validation processes to ensure that AI systems are built on a solid foundation.
Furthermore, the dynamic nature of data poses another challenge to maintaining data quality. As the world changes, so do the patterns and trends within data. AI models trained on static datasets may become obsolete over time, leading to outdated or irrelevant predictions. Continuous monitoring and updating of datasets are therefore necessary to ensure that AI systems remain accurate and unbiased.
To mitigate the risks associated with poor data quality, organizations must adopt robust data management practices. This includes implementing rigorous data cleaning and preprocessing techniques to eliminate errors and inconsistencies. Additionally, fostering a culture of transparency and accountability in AI development can help identify and address potential biases early in the process. Engaging diverse teams in the design and evaluation of AI systems can also provide valuable perspectives and insights, reducing the likelihood of biased outcomes.
In conclusion, the quality of data used in AI systems is a critical factor that can significantly influence their performance and fairness. Poor data quality can lead to biased results, perpetuating existing inequalities and undermining trust in AI technologies. By prioritizing data quality and adopting comprehensive data management strategies, organizations can build more reliable and equitable AI systems. As AI continues to play an increasingly prominent role in decision-making processes, ensuring the integrity of the data that fuels these systems is not just a technical challenge but a moral imperative.
Tools and Techniques for Ensuring High-Quality Data in AI Projects
In the rapidly evolving landscape of artificial intelligence, the quality of data used in AI projects is paramount. As AI systems become increasingly sophisticated, the demand for high-quality data has never been more critical. Poor data quality can lead to inaccurate models, biased outcomes, and ultimately, a lack of trust in AI systems. Therefore, ensuring high-quality data is essential for the success of any AI project. To achieve this, various tools and techniques have been developed to address the challenges associated with data quality.
One of the primary techniques for ensuring data quality is data cleaning. This process involves identifying and correcting errors or inconsistencies in the dataset. Data cleaning tools can automatically detect and rectify issues such as missing values, duplicate entries, and outliers. By employing these tools, data scientists can ensure that the dataset is accurate and reliable, which is crucial for training effective AI models. Moreover, data cleaning not only improves the quality of the data but also enhances the efficiency of the AI system by reducing the noise that can obscure meaningful patterns.
In addition to data cleaning, data validation is another critical technique for maintaining data quality. Data validation involves checking the dataset against predefined rules or constraints to ensure its integrity. This process can be automated using various software tools that can quickly identify and flag any data points that do not conform to the expected standards. By implementing data validation, organizations can prevent erroneous data from entering the system, thereby safeguarding the AI model from potential inaccuracies.
Furthermore, data augmentation is a valuable technique that can enhance the quality of data used in AI projects. Data augmentation involves generating new data points from the existing dataset through various transformations such as rotation, scaling, or flipping. This technique is particularly useful in scenarios where the dataset is limited or imbalanced. By augmenting the data, AI practitioners can create a more diverse and representative dataset, which can lead to more robust and generalizable models.
Another important aspect of ensuring data quality is the use of data governance frameworks. These frameworks provide a structured approach to managing data assets, ensuring that data is collected, stored, and processed in a consistent and compliant manner. Data governance frameworks establish clear guidelines and policies for data quality, security, and privacy, which are essential for maintaining the integrity of AI systems. By adhering to these frameworks, organizations can ensure that their data is not only high-quality but also ethically sourced and managed.
Moreover, leveraging advanced analytics and machine learning techniques can further enhance data quality. For instance, anomaly detection algorithms can automatically identify unusual patterns or deviations in the dataset, allowing for timely intervention and correction. Similarly, machine learning models can be trained to predict and fill in missing values, thereby improving the completeness of the dataset. These advanced techniques provide powerful tools for maintaining and improving data quality in AI projects.
In conclusion, the success of AI projects hinges on the quality of the data used. By employing a combination of data cleaning, validation, augmentation, governance, and advanced analytics, organizations can ensure that their data is of the highest quality. These tools and techniques not only enhance the accuracy and reliability of AI models but also build trust in AI systems by delivering consistent and unbiased outcomes. As AI continues to permeate various sectors, prioritizing data quality will remain a critical factor in harnessing the full potential of artificial intelligence.
Q&A
1. **What is data quality in AI?**
Data quality in AI refers to the accuracy, completeness, consistency, and reliability of data used to train and evaluate AI models. High-quality data ensures that AI systems perform effectively and make accurate predictions.
2. **Why is data quality important for AI?**
Data quality is crucial for AI because poor-quality data can lead to biased, inaccurate, or unreliable model outputs. High-quality data helps in building robust models that generalize well to new, unseen data.
3. **What are common signs of inadequate data quality in AI?**
Common signs include inconsistent model performance, unexpected biases in predictions, high error rates, and difficulty in model convergence during training.
4. **How can inadequate data quality affect AI outcomes?**
Inadequate data quality can result in AI models that produce incorrect or misleading results, leading to poor decision-making and potential negative impacts on business operations or user trust.
5. **What are some methods to improve data quality for AI?**
Methods include data cleaning (removing duplicates and errors), data augmentation (increasing dataset size and diversity), ensuring data consistency, and implementing robust data validation processes.
6. **How can organizations assess their data quality for AI projects?**
Organizations can assess data quality by conducting data audits, using data profiling tools, evaluating data sources, and continuously monitoring data quality metrics throughout the AI project lifecycle.Inadequate data quality can significantly impair the performance and reliability of AI systems. Poor data quality can lead to biased, inaccurate, or incomplete models, which in turn can result in flawed decision-making and reduced trust in AI applications. Ensuring high-quality data is crucial for training effective AI models, as it enhances their ability to generalize, improves accuracy, and ensures fairness. Organizations must prioritize data quality management, including data cleaning, validation, and enrichment processes, to mitigate risks and maximize the potential of their AI systems. Ultimately, investing in robust data quality practices is essential for the successful deployment and operation of AI technologies.
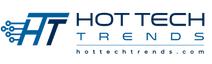