Artificial Intelligence (AI) has increasingly become a pivotal force in predicting and shaping future trends across various industries. One of the most promising advancements in this domain is the development of AI-driven graph models designed to predict innovation trends. These sophisticated models leverage the power of graph theory and machine learning to analyze complex networks of data, identifying patterns and relationships that are often invisible to traditional analytical methods. By mapping out the intricate web of connections between different technological domains, research papers, patents, and market dynamics, AI-driven graph models can forecast emerging trends and potential breakthroughs with remarkable accuracy. This capability not only aids businesses and policymakers in strategic decision-making but also accelerates the pace of innovation by highlighting areas ripe for exploration and investment. As these models continue to evolve, they hold the potential to transform how we understand and anticipate the future landscape of technology and innovation.
Understanding AI-Driven Graph Models in Predicting Innovation Trends
In the rapidly evolving landscape of technology and innovation, the ability to predict future trends is invaluable. One of the most promising tools in this endeavor is the AI-driven graph model, a sophisticated approach that leverages the power of artificial intelligence to analyze complex networks of information. These models are designed to identify patterns and relationships within vast datasets, offering insights that can guide strategic decision-making and foster innovation.
At the core of AI-driven graph models is the concept of graph theory, a branch of mathematics that studies the relationships between objects. In these models, data points are represented as nodes, while the connections between them are depicted as edges. This structure allows for a visual and analytical representation of complex systems, making it easier to discern patterns that might otherwise remain hidden. By applying AI algorithms to these graphs, it becomes possible to predict how certain trends might evolve over time.
One of the key advantages of using AI-driven graph models in predicting innovation trends is their ability to process and analyze large volumes of data with remarkable speed and accuracy. Traditional methods of trend analysis often rely on linear models that can be limited in scope and depth. In contrast, graph models can incorporate a multitude of variables and dimensions, providing a more comprehensive view of the innovation landscape. This capability is particularly useful in fields such as technology, where the pace of change is rapid and multifaceted.
Moreover, AI-driven graph models excel in identifying not only direct relationships but also indirect connections that may influence innovation trends. For instance, a new technological development in one sector might have ripple effects across various industries. By mapping these connections, graph models can highlight potential areas of opportunity or risk that might not be immediately apparent. This holistic approach enables businesses and policymakers to make more informed decisions, aligning their strategies with emerging trends.
In addition to their analytical prowess, AI-driven graph models are also highly adaptable. As new data becomes available, these models can be updated and refined, ensuring that predictions remain relevant and accurate. This dynamic nature is crucial in a world where information is constantly changing and evolving. Furthermore, the integration of machine learning techniques allows these models to improve over time, learning from past data to enhance future predictions.
Despite their many advantages, it is important to acknowledge the challenges associated with AI-driven graph models. One of the primary concerns is the quality and reliability of the data being used. Inaccurate or incomplete data can lead to flawed predictions, underscoring the need for robust data collection and validation processes. Additionally, the complexity of these models can make them difficult to interpret, necessitating a level of expertise that may not be readily available in all organizations.
Nevertheless, the potential of AI-driven graph models in predicting innovation trends is undeniable. As these models continue to evolve and improve, they are likely to become an indispensable tool for businesses and researchers alike. By providing a deeper understanding of the intricate web of relationships that drive innovation, AI-driven graph models offer a glimpse into the future, empowering decision-makers to navigate the complexities of the modern world with greater confidence and foresight.
The Role of Machine Learning in Innovation Trend Forecasting
In recent years, the rapid advancement of artificial intelligence (AI) has significantly transformed various sectors, including the field of innovation trend forecasting. One of the most promising developments in this area is the use of AI-driven graph models, which have emerged as powerful tools for predicting innovation trends. These models leverage the vast amounts of data generated in today’s digital age, enabling organizations to anticipate future developments and make informed strategic decisions. As we delve into the role of machine learning in innovation trend forecasting, it is essential to understand how these graph models function and the benefits they offer.
At the core of AI-driven graph models is the ability to represent complex relationships between different entities, such as technologies, companies, and research papers, in a structured manner. By mapping these relationships, graph models can identify patterns and connections that may not be immediately apparent through traditional analysis methods. This capability is particularly valuable in the context of innovation, where the interplay between various factors can be intricate and multifaceted. Machine learning algorithms, when applied to these graph structures, can uncover hidden insights and predict emerging trends with remarkable accuracy.
One of the key advantages of using AI-driven graph models in innovation trend forecasting is their ability to process and analyze large datasets efficiently. In an era where data is generated at an unprecedented rate, the capacity to handle vast amounts of information is crucial. Graph models excel in this regard, as they can integrate diverse data sources, such as patent filings, academic publications, and market reports, into a cohesive framework. This integration allows for a comprehensive analysis of the innovation landscape, providing a holistic view of current trends and potential future directions.
Moreover, AI-driven graph models are adept at capturing the dynamic nature of innovation. Unlike static models, which may struggle to adapt to rapidly changing environments, graph models can continuously update and refine their predictions as new data becomes available. This adaptability ensures that organizations remain at the forefront of innovation, enabling them to respond swiftly to emerging opportunities and challenges. Furthermore, the predictive capabilities of these models can be enhanced through the incorporation of advanced machine learning techniques, such as deep learning and reinforcement learning, which further improve their accuracy and reliability.
In addition to their technical prowess, AI-driven graph models offer significant strategic benefits for organizations seeking to foster innovation. By providing insights into potential future trends, these models enable companies to allocate resources more effectively, prioritize research and development efforts, and identify potential collaborators or competitors. This strategic foresight can be a critical differentiator in today’s competitive landscape, where the ability to innovate rapidly and effectively is often a key determinant of success.
As we consider the role of machine learning in innovation trend forecasting, it is clear that AI-driven graph models represent a significant advancement in this field. Their ability to process large datasets, capture dynamic relationships, and provide strategic insights makes them invaluable tools for organizations looking to stay ahead of the curve. As technology continues to evolve, it is likely that these models will become even more sophisticated, further enhancing their predictive capabilities and solidifying their role in shaping the future of innovation. In conclusion, the integration of AI-driven graph models into innovation trend forecasting marks a pivotal step forward, offering a glimpse into a future where data-driven insights drive strategic decision-making and fuel the next wave of technological advancements.
How Graph Models Enhance Predictive Analytics for Innovation
In the rapidly evolving landscape of technology and innovation, the ability to predict future trends is invaluable. Organizations and researchers are increasingly turning to advanced analytical tools to gain insights into the trajectory of innovation. Among these tools, AI-driven graph models have emerged as a powerful method for enhancing predictive analytics. These models leverage the interconnected nature of data to provide a more comprehensive understanding of potential innovation trends.
Graph models, at their core, represent data as a network of nodes and edges, where nodes signify entities and edges denote the relationships between them. This structure is particularly well-suited for capturing the complex, interrelated nature of innovation ecosystems. By utilizing graph models, analysts can map out the intricate web of relationships that exist between various entities, such as companies, technologies, and research institutions. This mapping allows for a more nuanced analysis of how innovations emerge and evolve over time.
One of the primary advantages of using graph models in predictive analytics is their ability to incorporate a wide range of data sources. Traditional models often rely on linear or isolated datasets, which can limit their predictive power. In contrast, graph models can integrate diverse data types, including patent filings, academic publications, and market data, to create a holistic view of the innovation landscape. This integration enables the identification of patterns and trends that might otherwise remain hidden in siloed datasets.
Moreover, AI-driven graph models are equipped with machine learning algorithms that can process and analyze vast amounts of data with remarkable speed and accuracy. These algorithms can identify subtle patterns and correlations within the data, providing insights that are both deep and actionable. For instance, by analyzing the connections between different technologies and their respective development trajectories, graph models can predict which technologies are likely to converge and lead to groundbreaking innovations.
In addition to identifying potential innovation trends, graph models can also assess the impact of these trends on various industries. By simulating different scenarios and analyzing the potential outcomes, organizations can make informed decisions about where to allocate resources and how to position themselves in the market. This capability is particularly valuable in industries characterized by rapid technological change, where staying ahead of the curve is crucial for maintaining a competitive edge.
Furthermore, the use of graph models in predictive analytics is not limited to large corporations or research institutions. With the increasing availability of open-source graph analytics tools and platforms, smaller organizations and startups can also leverage this technology to gain insights into innovation trends. This democratization of access to advanced analytical tools is fostering a more inclusive innovation ecosystem, where a wider range of players can contribute to and benefit from technological advancements.
In conclusion, AI-driven graph models represent a significant advancement in the field of predictive analytics for innovation. By capturing the complex relationships within innovation ecosystems and integrating diverse data sources, these models provide a more comprehensive and accurate picture of future trends. As technology continues to evolve, the role of graph models in predicting innovation trends is likely to become even more prominent, offering valuable insights that can drive strategic decision-making and foster a more dynamic and competitive innovation landscape.
Case Studies: Successful Innovation Predictions Using AI-Driven Graph Models
In recent years, the integration of artificial intelligence (AI) into various sectors has revolutionized the way industries predict and adapt to emerging trends. One of the most compelling applications of AI is in the realm of innovation prediction, where AI-driven graph models have demonstrated remarkable success. These models, which leverage the interconnectedness of data points, offer a sophisticated means of forecasting future trends by analyzing complex relationships within vast datasets. This case study explores several instances where AI-driven graph models have successfully predicted innovation trends, thereby providing valuable insights for businesses and researchers alike.
To begin with, it is essential to understand the fundamental workings of AI-driven graph models. These models utilize graph theory, a branch of mathematics that studies the relationships between objects. By representing data as nodes and the connections between them as edges, graph models can capture intricate patterns and dependencies that traditional linear models might overlook. This capability is particularly advantageous in predicting innovation trends, where the interplay between various factors can be highly complex and non-linear.
One notable case study involves the pharmaceutical industry, where AI-driven graph models have been employed to predict the development of new drugs. By analyzing vast amounts of biomedical data, including research papers, clinical trial results, and patent filings, these models can identify potential areas of innovation. For instance, a graph model might reveal a previously unnoticed connection between a particular protein and a disease, suggesting a new target for drug development. In one instance, a pharmaceutical company used such a model to predict the emergence of a novel class of antibiotics, which subsequently led to successful drug development and commercialization.
Transitioning to another sector, the technology industry has also benefited significantly from AI-driven graph models. In this fast-paced field, staying ahead of innovation trends is crucial for maintaining a competitive edge. Companies have utilized graph models to analyze data from patent databases, research publications, and market trends to forecast technological advancements. For example, a leading tech firm employed a graph model to predict the rise of quantum computing technologies. By identifying key research clusters and their interconnections, the model provided insights into which areas were likely to experience breakthroughs, allowing the company to strategically invest in relevant research and development initiatives.
Moreover, the field of renewable energy has seen successful applications of AI-driven graph models in predicting innovation trends. As the world increasingly shifts towards sustainable energy solutions, understanding the trajectory of technological advancements in this area is vital. Graph models have been used to analyze data from scientific literature, industry reports, and government policies to forecast developments in solar and wind energy technologies. In one case, a renewable energy company utilized a graph model to predict advancements in solar panel efficiency, enabling them to optimize their production processes and gain a competitive advantage in the market.
In conclusion, AI-driven graph models have proven to be powerful tools in predicting innovation trends across various industries. By capturing complex relationships within data, these models provide valuable insights that can guide strategic decision-making and investment. As AI technology continues to evolve, it is likely that the application of graph models in innovation prediction will become even more sophisticated, offering unprecedented opportunities for businesses and researchers to anticipate and shape the future. Through these case studies, it is evident that AI-driven graph models are not only enhancing our understanding of innovation but also paving the way for a more informed and proactive approach to technological advancement.
Challenges and Opportunities in AI-Driven Innovation Trend Prediction
The advent of artificial intelligence (AI) has revolutionized numerous fields, and its application in predicting innovation trends is no exception. AI-driven graph models, in particular, have emerged as powerful tools in this domain, offering unprecedented insights into the complex web of technological advancements and market dynamics. However, while these models present significant opportunities, they also come with a set of challenges that must be addressed to fully harness their potential.
One of the primary challenges in AI-driven innovation trend prediction is the sheer volume and complexity of data involved. Innovation is influenced by a multitude of factors, including technological developments, market demands, regulatory changes, and socio-economic trends. AI-driven graph models must process and analyze vast amounts of data from diverse sources to identify meaningful patterns and trends. This requires sophisticated algorithms capable of handling high-dimensional data and extracting relevant information without being overwhelmed by noise.
Moreover, the dynamic nature of innovation poses another challenge. Technological advancements and market conditions are constantly evolving, making it difficult for static models to provide accurate predictions over time. To address this, AI-driven graph models must be designed to adapt and learn continuously from new data. This involves implementing machine learning techniques that allow the models to update their predictions as new information becomes available, ensuring that they remain relevant and accurate in a rapidly changing environment.
Despite these challenges, the opportunities presented by AI-driven graph models in predicting innovation trends are substantial. By leveraging the power of AI, organizations can gain valuable foresight into emerging technologies and market shifts, enabling them to make informed strategic decisions. For instance, companies can identify potential areas for investment, anticipate changes in consumer preferences, and develop innovative products and services that align with future trends. This proactive approach can provide a significant competitive advantage in today’s fast-paced business landscape.
Furthermore, AI-driven graph models can facilitate collaboration and knowledge sharing across industries. By mapping the relationships between different technologies and market trends, these models can help identify synergies and opportunities for cross-industry innovation. This can lead to the development of novel solutions that address complex challenges and create new value for businesses and consumers alike.
In addition to these opportunities, AI-driven graph models also have the potential to democratize access to innovation insights. Traditionally, predicting innovation trends has been the domain of large corporations with significant resources and expertise. However, AI-driven models can level the playing field by providing smaller organizations and startups with access to the same insights and predictive capabilities. This can foster a more inclusive and diverse innovation ecosystem, where a wider range of players can contribute to and benefit from technological advancements.
In conclusion, while AI-driven graph models offer significant opportunities for predicting innovation trends, they also present a set of challenges that must be addressed. By developing sophisticated algorithms capable of handling complex data and adapting to dynamic environments, organizations can harness the power of AI to gain valuable insights into the future of innovation. This not only provides a competitive advantage but also fosters collaboration and inclusivity in the innovation ecosystem. As AI technology continues to evolve, it is likely that these models will become even more integral to the process of predicting and shaping the future of innovation.
Future Prospects of AI-Driven Graph Models in Shaping Innovation Strategies
In the rapidly evolving landscape of technology, the ability to predict innovation trends has become a crucial asset for businesses and policymakers alike. The advent of AI-driven graph models marks a significant advancement in this domain, offering unprecedented insights into the complex web of factors that drive innovation. These models leverage the power of artificial intelligence to analyze vast amounts of data, identifying patterns and relationships that would be impossible for humans to discern unaided. As a result, they provide a more nuanced understanding of how innovations emerge, evolve, and impact various sectors.
AI-driven graph models operate by representing data as nodes and edges, where nodes signify entities such as companies, technologies, or patents, and edges represent the relationships between them. This structure allows for a dynamic and interconnected view of the innovation ecosystem. By applying machine learning algorithms to these graphs, it becomes possible to predict future trends with remarkable accuracy. For instance, these models can identify which technologies are likely to converge, which companies are poised to lead in specific domains, and how shifts in one area might ripple through the entire network.
The implications of these capabilities are profound. For businesses, AI-driven graph models can inform strategic decisions by highlighting emerging opportunities and potential threats. Companies can use these insights to allocate resources more effectively, prioritize research and development efforts, and forge strategic partnerships. Moreover, by anticipating shifts in consumer preferences and technological advancements, businesses can stay ahead of the competition and maintain a competitive edge.
Policymakers, too, stand to benefit from the predictive power of AI-driven graph models. By understanding the trajectories of innovation, governments can craft policies that foster sustainable growth and address societal challenges. For example, by identifying sectors with high innovation potential, policymakers can direct funding and support to areas that promise the greatest economic and social returns. Additionally, these models can help anticipate the societal impacts of new technologies, enabling proactive measures to mitigate potential risks.
Despite their promise, the deployment of AI-driven graph models is not without challenges. One significant hurdle is the quality and availability of data. For these models to function effectively, they require access to comprehensive and up-to-date datasets. Ensuring data privacy and security is another critical concern, as the sensitive nature of the information involved necessitates robust safeguards. Furthermore, the complexity of these models demands a high level of expertise to develop and interpret, which may limit their accessibility to larger organizations with the necessary resources.
Nevertheless, ongoing advancements in AI and data science are likely to address many of these challenges, making AI-driven graph models increasingly accessible and effective. As these models continue to evolve, they will undoubtedly play a pivotal role in shaping innovation strategies across industries. By providing a clearer picture of the innovation landscape, they empower decision-makers to navigate the complexities of the modern world with greater confidence and foresight.
In conclusion, AI-driven graph models represent a transformative tool in the quest to understand and predict innovation trends. Their ability to analyze complex networks of data offers valuable insights that can guide strategic decision-making for businesses and policymakers alike. As technology continues to advance, the role of these models in shaping the future of innovation is poised to grow, heralding a new era of informed and strategic innovation management.
Q&A
1. **What is an AI-driven graph model?**
An AI-driven graph model is a computational framework that uses artificial intelligence to analyze and interpret data structured as graphs, which consist of nodes (entities) and edges (relationships). This model can identify patterns and insights from complex interconnected data.
2. **How does an AI-driven graph model predict innovation trends?**
It predicts innovation trends by analyzing large datasets of patents, research papers, and other innovation-related documents. The model identifies emerging patterns, relationships, and clusters of activity that indicate new areas of technological development and potential future innovations.
3. **What data sources are typically used in these models?**
Common data sources include patent databases, academic publications, industry reports, and market analysis documents. These sources provide the raw data needed to map out the innovation landscape and identify trends.
4. **What are the benefits of using AI-driven graph models for predicting innovation?**
Benefits include the ability to process and analyze vast amounts of data quickly, uncover hidden connections and trends, provide data-driven insights for strategic decision-making, and enhance the ability to forecast future technological developments.
5. **What industries can benefit from AI-driven graph models predicting innovation trends?**
Industries such as technology, pharmaceuticals, automotive, finance, and manufacturing can benefit. These models help companies stay ahead of the competition by identifying emerging technologies and market opportunities.
6. **What challenges exist in using AI-driven graph models for innovation prediction?**
Challenges include ensuring data quality and completeness, managing the complexity of large and dynamic datasets, addressing privacy and ethical concerns, and the need for continuous model updates to reflect the latest data and trends.AI-driven graph models are increasingly being utilized to predict innovation trends by analyzing complex relationships and patterns within vast datasets. These models leverage the interconnected nature of data, capturing insights from various domains such as patents, research publications, and market dynamics. By identifying emerging technologies, key influencers, and potential collaboration opportunities, AI-driven graph models provide valuable foresight into future innovation trajectories. This predictive capability enables businesses, researchers, and policymakers to make informed decisions, allocate resources effectively, and maintain a competitive edge in rapidly evolving industries. Ultimately, AI-driven graph models represent a powerful tool for anticipating and shaping the future landscape of innovation.
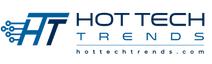