GraphCast: Revolutionizing Global Weather Forecasting with AI Precision
In the rapidly evolving field of meteorology, GraphCast emerges as a groundbreaking innovation, harnessing the power of artificial intelligence to transform global weather forecasting. By integrating advanced machine learning algorithms with vast datasets, GraphCast offers unprecedented accuracy and precision in predicting weather patterns. This cutting-edge technology not only enhances the reliability of forecasts but also provides critical insights for industries ranging from agriculture to aviation. As climate change continues to challenge traditional forecasting methods, GraphCast stands at the forefront, delivering timely and precise weather predictions that empower decision-makers worldwide.
Understanding GraphCast: The Future of Weather Forecasting
GraphCast represents a significant leap forward in the realm of weather forecasting, harnessing the power of artificial intelligence to deliver unprecedented precision and reliability. As climate change continues to alter weather patterns globally, the need for accurate and timely weather predictions has never been more critical. Traditional forecasting methods, while effective to a degree, often struggle with the complexity and variability inherent in atmospheric systems. This is where GraphCast steps in, offering a transformative approach that leverages advanced AI algorithms to enhance our understanding and prediction of weather phenomena.
At the core of GraphCast’s innovation is its use of graph neural networks, a sophisticated form of AI that excels in processing data with intricate relationships. Unlike conventional neural networks, which operate on fixed-size input data, graph neural networks can handle data that is structured as graphs, making them particularly adept at modeling the interconnected nature of atmospheric variables. This capability allows GraphCast to integrate vast amounts of meteorological data from diverse sources, such as satellite imagery, ground-based sensors, and historical weather records, into a cohesive and dynamic model of the Earth’s atmosphere.
Moreover, GraphCast’s AI-driven approach enables it to learn and adapt continuously, improving its predictive accuracy over time. By analyzing patterns and correlations within the data, GraphCast can identify subtle signals that might be overlooked by traditional models. This results in forecasts that are not only more accurate but also more granular, providing detailed insights into localized weather events. For instance, GraphCast can predict the onset and trajectory of severe weather conditions, such as hurricanes or thunderstorms, with greater precision, allowing for more effective disaster preparedness and response.
In addition to its technical prowess, GraphCast also offers significant practical benefits. Its ability to deliver real-time forecasts with high accuracy can revolutionize various sectors, from agriculture to aviation. Farmers can optimize their planting and harvesting schedules based on precise weather predictions, thereby enhancing crop yields and reducing losses. Similarly, airlines can improve flight safety and efficiency by anticipating and mitigating weather-related disruptions. Furthermore, GraphCast’s insights can aid policymakers in developing more informed strategies for climate adaptation and resilience, ultimately contributing to the protection of communities and ecosystems.
Transitioning from traditional methods to AI-driven forecasting, however, is not without its challenges. The integration of GraphCast into existing meteorological frameworks requires careful consideration of data privacy, computational resources, and the need for continuous validation and verification of its predictions. Nevertheless, the potential benefits far outweigh these hurdles, as GraphCast promises to redefine the standards of accuracy and reliability in weather forecasting.
In conclusion, GraphCast stands at the forefront of a new era in meteorology, where artificial intelligence plays a pivotal role in understanding and predicting the complexities of our planet’s weather systems. By combining cutting-edge technology with vast datasets, GraphCast not only enhances our ability to forecast weather with remarkable precision but also empowers various industries and communities to make informed decisions in the face of an ever-changing climate. As we continue to refine and expand the capabilities of AI in weather forecasting, GraphCast exemplifies the transformative potential of technology in addressing one of humanity’s most enduring challenges.
How AI Precision in GraphCast Enhances Weather Predictions
GraphCast, a groundbreaking innovation in the realm of meteorology, is transforming the way we understand and predict weather patterns across the globe. By harnessing the power of artificial intelligence, GraphCast offers unprecedented precision in weather forecasting, marking a significant leap forward from traditional methods. This advancement is not merely a technological feat but a crucial development with far-reaching implications for various sectors, including agriculture, disaster management, and transportation.
At the core of GraphCast’s success is its utilization of AI-driven algorithms that process vast amounts of meteorological data with remarkable speed and accuracy. Unlike conventional models that rely heavily on numerical weather prediction, GraphCast employs machine learning techniques to analyze complex datasets. This approach allows for the identification of intricate patterns and relationships within the data that might otherwise go unnoticed. Consequently, GraphCast can generate forecasts that are not only more accurate but also more reliable over extended periods.
One of the key advantages of GraphCast is its ability to integrate diverse data sources, ranging from satellite imagery to ground-based observations. By synthesizing this information, the system creates a comprehensive picture of atmospheric conditions, enabling it to make more informed predictions. Furthermore, the AI algorithms continuously learn and adapt, refining their predictive capabilities as new data becomes available. This dynamic learning process ensures that GraphCast remains at the forefront of meteorological forecasting, consistently improving its performance.
In addition to its technical prowess, GraphCast’s precision in weather forecasting has significant practical benefits. For instance, in agriculture, accurate weather predictions are essential for optimizing planting and harvesting schedules, thereby enhancing crop yields and reducing waste. Similarly, in disaster management, timely and precise forecasts can be the difference between life and death. By providing early warnings of severe weather events such as hurricanes and floods, GraphCast enables authorities to implement evacuation plans and other safety measures more effectively.
Moreover, the transportation industry stands to gain immensely from GraphCast’s capabilities. With precise weather forecasts, airlines can optimize flight routes to avoid turbulence and adverse weather conditions, leading to safer and more efficient air travel. Similarly, shipping companies can plan their routes to minimize the impact of storms and other weather-related disruptions, ensuring timely delivery of goods.
Despite these advantages, the implementation of AI in weather forecasting is not without challenges. Ensuring the accuracy and reliability of AI models requires continuous validation and verification against real-world observations. Additionally, there is a need for collaboration between meteorologists and data scientists to interpret AI-generated forecasts effectively. By addressing these challenges, the potential of GraphCast can be fully realized, paving the way for even more sophisticated forecasting systems in the future.
In conclusion, GraphCast represents a significant advancement in the field of weather forecasting, offering unparalleled precision through the integration of artificial intelligence. Its ability to process and analyze vast amounts of data with speed and accuracy has far-reaching implications for various sectors, enhancing decision-making and improving safety and efficiency. As AI technology continues to evolve, GraphCast is poised to lead the charge in revolutionizing global weather forecasting, setting a new standard for accuracy and reliability in the years to come.
The Impact of GraphCast on Global Climate Monitoring
GraphCast, an innovative artificial intelligence (AI) system, is poised to transform global climate monitoring by enhancing the precision and reliability of weather forecasts. As climate change continues to pose significant challenges worldwide, the need for accurate and timely weather predictions has never been more critical. Traditional forecasting methods, while effective to a certain extent, often struggle with the complexity and variability inherent in global weather patterns. This is where GraphCast steps in, offering a sophisticated solution that leverages the power of AI to provide more accurate and detailed forecasts.
At the core of GraphCast’s revolutionary approach is its ability to process vast amounts of data with remarkable speed and accuracy. Unlike conventional models that rely heavily on numerical weather prediction, GraphCast utilizes advanced machine learning algorithms to analyze and interpret complex datasets. This enables the system to identify patterns and trends that might otherwise go unnoticed, thereby improving the accuracy of forecasts. Furthermore, GraphCast’s AI-driven methodology allows it to continuously learn and adapt, refining its predictions as new data becomes available. This dynamic capability ensures that the system remains at the forefront of weather forecasting technology.
In addition to its enhanced predictive capabilities, GraphCast also offers significant advantages in terms of efficiency and scalability. Traditional weather models often require substantial computational resources and time to generate forecasts, which can limit their effectiveness in rapidly changing conditions. GraphCast, on the other hand, is designed to operate with greater efficiency, delivering accurate forecasts in a fraction of the time. This not only allows for more timely dissemination of critical weather information but also enables the system to scale effectively, providing reliable forecasts across diverse geographical regions.
Moreover, the impact of GraphCast extends beyond mere weather prediction. By offering more precise and timely forecasts, the system plays a crucial role in global climate monitoring efforts. Accurate weather data is essential for understanding and mitigating the effects of climate change, as it informs decision-making processes related to disaster preparedness, resource management, and environmental conservation. GraphCast’s ability to deliver high-quality forecasts thus contributes significantly to these efforts, supporting initiatives aimed at safeguarding communities and ecosystems worldwide.
Furthermore, the integration of GraphCast into existing climate monitoring frameworks has the potential to enhance collaboration and data sharing among international organizations. By providing a common platform for accessing reliable weather information, GraphCast facilitates greater cooperation and coordination in addressing global climate challenges. This collaborative approach is essential for developing comprehensive strategies to combat the adverse effects of climate change and promote sustainable development.
In conclusion, GraphCast represents a significant advancement in the field of global weather forecasting and climate monitoring. Its innovative use of AI technology not only improves the accuracy and efficiency of weather predictions but also supports broader efforts to address the impacts of climate change. As the world continues to grapple with the challenges posed by a changing climate, systems like GraphCast will play an increasingly vital role in ensuring that communities and ecosystems are better prepared and more resilient. Through its contributions to global climate monitoring, GraphCast exemplifies the transformative potential of AI in addressing some of the most pressing issues of our time.
GraphCast vs. Traditional Weather Forecasting Methods
In the realm of meteorology, the advent of GraphCast represents a significant leap forward in weather forecasting, offering a stark contrast to traditional methods. Traditional weather forecasting has long relied on numerical weather prediction (NWP) models, which use mathematical equations to simulate atmospheric processes. These models, while effective, are computationally intensive and often require significant time to produce forecasts. They depend heavily on initial conditions and can be limited by the resolution of the data they process. As a result, traditional methods sometimes struggle with accuracy, particularly in predicting extreme weather events or rapidly changing conditions.
Enter GraphCast, an innovative approach that leverages the power of artificial intelligence to enhance the precision and efficiency of weather forecasts. Unlike traditional models, GraphCast utilizes graph neural networks, a type of AI that excels in processing data with complex relationships. This allows GraphCast to analyze vast amounts of meteorological data more quickly and accurately than conventional methods. By understanding the intricate web of interactions within the atmosphere, GraphCast can generate forecasts with a higher degree of precision, even in scenarios where traditional models might falter.
One of the key advantages of GraphCast over traditional methods is its ability to process data in real-time. Traditional NWP models often require hours to run, which can delay the dissemination of critical weather information. In contrast, GraphCast’s AI-driven approach can produce forecasts in a fraction of the time, enabling meteorologists to provide timely updates and warnings. This rapid processing capability is particularly beneficial in situations where weather conditions are changing rapidly, such as during the development of severe storms or hurricanes.
Moreover, GraphCast’s AI algorithms are designed to learn and improve over time. As they are exposed to more data, these algorithms refine their understanding of atmospheric patterns, leading to progressively more accurate forecasts. This self-improving feature is a significant departure from traditional models, which require manual adjustments and recalibrations to enhance their performance. Consequently, GraphCast not only offers immediate improvements in forecasting accuracy but also promises continued advancements as it evolves.
Another notable distinction between GraphCast and traditional methods is the former’s ability to integrate diverse data sources seamlessly. Traditional models often rely on a limited set of data inputs, such as satellite imagery and ground-based observations. GraphCast, however, can incorporate a broader range of data, including social media feeds, IoT sensors, and other unconventional sources. This comprehensive data integration allows GraphCast to capture a more holistic view of the atmospheric conditions, further enhancing its forecasting capabilities.
Despite these advantages, it is important to acknowledge that GraphCast is not without its challenges. The reliance on AI and machine learning requires robust computational infrastructure and expertise in data science, which may not be readily available in all meteorological institutions. Additionally, the transition from traditional methods to AI-driven forecasting necessitates a paradigm shift in how meteorologists approach their work, requiring training and adaptation.
In conclusion, GraphCast represents a transformative development in weather forecasting, offering significant improvements over traditional methods in terms of speed, accuracy, and adaptability. By harnessing the power of AI, GraphCast is poised to revolutionize the field, providing more reliable forecasts that can better inform decision-making and enhance public safety. As this technology continues to evolve, it holds the promise of further advancements, paving the way for a new era in meteorological science.
The Role of Machine Learning in GraphCast’s Success
GraphCast, a groundbreaking innovation in global weather forecasting, has emerged as a testament to the transformative power of machine learning. At the heart of GraphCast’s success lies its sophisticated use of machine learning algorithms, which have redefined the accuracy and efficiency of weather predictions. By leveraging the capabilities of artificial intelligence, GraphCast has set a new standard in meteorological science, offering unprecedented precision in forecasting weather patterns across the globe.
Machine learning, a subset of artificial intelligence, involves the development of algorithms that enable computers to learn from and make predictions based on data. In the context of GraphCast, machine learning plays a pivotal role in processing vast amounts of meteorological data, which includes temperature, humidity, wind patterns, and atmospheric pressure. These data points are collected from a myriad of sources, such as satellites, weather stations, and ocean buoys, creating a complex web of information that traditional forecasting methods struggle to analyze efficiently.
One of the key advantages of machine learning in GraphCast is its ability to identify patterns and correlations within this extensive dataset that may not be immediately apparent to human analysts. By employing advanced techniques such as neural networks and deep learning, GraphCast can discern subtle relationships between different meteorological variables, leading to more accurate predictions. For instance, machine learning models can detect early signs of severe weather events, such as hurricanes or tornadoes, by recognizing specific patterns in atmospheric data that precede these phenomena.
Moreover, the adaptability of machine learning algorithms is crucial to GraphCast’s success. Unlike static models, machine learning systems can continuously update and refine their predictions as new data becomes available. This dynamic approach allows GraphCast to provide real-time updates and adjust forecasts with remarkable speed and precision. Consequently, this capability is particularly beneficial in rapidly changing weather conditions, where timely and accurate information is essential for decision-making processes in sectors such as agriculture, aviation, and disaster management.
In addition to enhancing the accuracy of weather forecasts, machine learning also contributes to the efficiency of GraphCast’s operations. Traditional weather forecasting methods often require significant computational resources and time to process data and generate predictions. In contrast, machine learning algorithms can streamline these processes by automating data analysis and reducing the computational burden. This efficiency not only accelerates the forecasting process but also enables GraphCast to allocate resources more effectively, focusing on refining models and expanding their predictive capabilities.
Furthermore, the integration of machine learning into GraphCast has facilitated the development of user-friendly interfaces and applications that make weather information more accessible to the public. By translating complex meteorological data into intuitive visualizations and actionable insights, GraphCast empowers individuals and organizations to make informed decisions based on reliable weather forecasts. This democratization of weather information underscores the broader societal impact of machine learning in enhancing public safety and economic resilience.
In conclusion, the role of machine learning in GraphCast’s success cannot be overstated. Through its ability to process vast datasets, identify intricate patterns, and adapt to new information, machine learning has revolutionized global weather forecasting. As GraphCast continues to evolve, the integration of machine learning will undoubtedly remain a cornerstone of its innovative approach, paving the way for even greater advancements in meteorological science and technology.
Real-World Applications of GraphCast in Weather Forecasting
GraphCast, an innovative artificial intelligence (AI) system, is transforming the landscape of global weather forecasting with unprecedented precision and efficiency. Developed to address the limitations of traditional meteorological models, GraphCast leverages advanced machine learning techniques to provide more accurate and timely weather predictions. This cutting-edge technology is not only enhancing the accuracy of forecasts but also offering significant benefits across various sectors that rely heavily on weather data.
At the core of GraphCast’s functionality is its ability to process vast amounts of meteorological data from diverse sources, including satellite imagery, ground-based observations, and historical weather patterns. By integrating these data points, GraphCast constructs a comprehensive and dynamic model of the Earth’s atmosphere. This model is continuously updated, allowing for real-time analysis and prediction of weather events. Unlike conventional models that often require extensive computational resources and time, GraphCast operates with remarkable speed and efficiency, delivering forecasts in a fraction of the time.
One of the most significant real-world applications of GraphCast is in the field of disaster management. Accurate and timely weather forecasts are crucial for preparing and responding to natural disasters such as hurricanes, floods, and wildfires. GraphCast’s ability to predict these events with greater precision enables authorities to implement more effective evacuation plans, allocate resources more efficiently, and ultimately save lives. For instance, during hurricane season, GraphCast can provide early warnings and detailed projections of storm paths, helping communities to better prepare and mitigate potential damage.
In addition to disaster management, GraphCast is proving invaluable in agriculture, where weather conditions play a critical role in crop yield and food security. Farmers can utilize GraphCast’s forecasts to make informed decisions about planting, irrigation, and harvesting, thereby optimizing their operations and reducing losses due to adverse weather. This application is particularly beneficial in regions where climate variability poses a significant challenge to agricultural productivity. By providing reliable weather predictions, GraphCast supports sustainable farming practices and contributes to global food security.
Furthermore, the transportation industry is reaping the benefits of GraphCast’s advanced forecasting capabilities. Airlines, shipping companies, and logistics providers rely on accurate weather data to ensure the safety and efficiency of their operations. GraphCast’s precise forecasts allow these industries to anticipate and mitigate weather-related disruptions, such as flight delays, route diversions, and supply chain interruptions. Consequently, this leads to improved operational efficiency, cost savings, and enhanced customer satisfaction.
Moreover, GraphCast’s impact extends to the energy sector, where weather conditions significantly influence energy production and consumption. Renewable energy sources, such as solar and wind, are particularly dependent on accurate weather forecasts to optimize energy generation and grid management. By providing detailed predictions of weather patterns, GraphCast enables energy providers to better balance supply and demand, reduce reliance on fossil fuels, and support the transition to a more sustainable energy future.
In conclusion, GraphCast is revolutionizing global weather forecasting by harnessing the power of AI to deliver precise and timely predictions. Its real-world applications are vast and varied, offering significant benefits across multiple sectors, including disaster management, agriculture, transportation, and energy. As climate change continues to pose challenges to traditional forecasting methods, GraphCast represents a promising solution that enhances our ability to understand and respond to the complexities of the Earth’s atmosphere. Through its innovative approach, GraphCast is setting a new standard for accuracy and efficiency in weather forecasting, ultimately contributing to a safer and more resilient world.
Q&A
1. **What is GraphCast?**
GraphCast is an AI-based system designed to enhance global weather forecasting by leveraging advanced machine learning techniques to improve prediction accuracy and efficiency.
2. **How does GraphCast improve weather forecasting?**
GraphCast utilizes graph neural networks to model complex atmospheric patterns, allowing it to process vast amounts of meteorological data and generate precise weather forecasts.
3. **What are the key features of GraphCast?**
Key features include high-resolution forecasts, rapid processing times, and the ability to integrate diverse data sources, such as satellite imagery and sensor data, to produce comprehensive weather models.
4. **Who developed GraphCast?**
GraphCast was developed by a team of researchers and engineers specializing in artificial intelligence and meteorology, often involving collaboration between tech companies and academic institutions.
5. **What are the benefits of using GraphCast over traditional methods?**
GraphCast offers improved accuracy, faster updates, and the ability to predict extreme weather events more reliably, which can lead to better preparedness and response strategies.
6. **How is GraphCast being implemented globally?**
GraphCast is being adopted by meteorological agencies and organizations worldwide, integrating into existing forecasting systems to enhance their capabilities and provide more reliable weather information to the public.GraphCast represents a significant advancement in global weather forecasting by leveraging AI precision to enhance prediction accuracy and efficiency. By utilizing advanced machine learning algorithms and graph neural networks, GraphCast can process vast amounts of meteorological data to generate highly detailed and reliable weather forecasts. This innovative approach not only improves the timeliness and precision of weather predictions but also offers the potential to better anticipate extreme weather events, thereby aiding in disaster preparedness and response. As a result, GraphCast is poised to revolutionize the field of meteorology, providing valuable insights and tools for both scientists and the general public, ultimately contributing to safer and more informed communities worldwide.
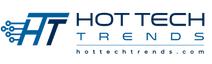